The Dawn of Artificial Imagination
OpenAI says programs like DALL-E 2 will “democratize” art.
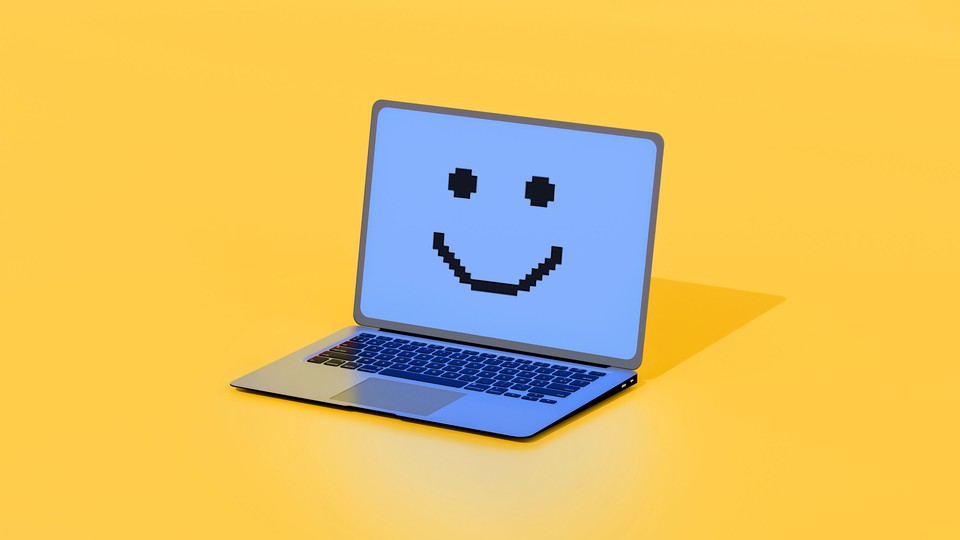
For years, fears about the disruptive potential of automation and artificial intelligence have centered on repetitive labor: Perhaps machines could replace humans who do secretarial work, accounting, burger-flipping. Doctors, software engineers, authors—any job that requires creative intelligence—seemed safe. But the past few months have turned those narratives on their head. A wave of artificial-intelligence programs, collectively dubbed “generative AI,” have shown remarkable aptitude at using the English language, competition-level coding, creating stunning images from simple prompts, and perhaps even helping discover new drugs. In a year that has seen numerous tech hype bubbles burst or deflate, these applications suggest that Silicon Valley still has the power to, in subtle and shocking ways, rewire the world.
A reasonable reaction to generative AI is concern; if not even the imagination is safe from machines, the human mind seems at risk of becoming obsolete. Another is to point to these algorithms’ many biases and shortcomings. But these new models also spark wonder, of a science-fictional variety—perhaps computers will not supersede human creativity so much as augment or transform it. Our brains have largely benefited from calculators, computers, and even internet search engines, after all.
“The reason we built this tool is to really democratize image generation for a bunch of people who wouldn’t necessarily classify themselves as artists,” Mark Chen, the lead researcher on DALL-E 2, a model from OpenAI that transforms written prompts into visual art, said during The Atlantic’s first-ever Progress Summit yesterday. “With AI, you always worry about job loss and displacement, and we don’t want to kind of ignore these possibilities either. But we do think it’s a tool that allows people to be creative, and we’ve seen, so far, artists are more creative with it than regular users. And there’s a lot of technologies like this—smartphone cameras haven’t replaced photographers.”
Chen was joined by The Atlantic’s deputy editor, Ross Andersen, for a wide-ranging conversation on the future of human creativity and artificial intelligence. They discussed how DALL-E 2 works, the pushback OpenAI has received from artists, and the implications of text-to-image programs for developing a more general artificial intelligence.
Their conversation has been edited and condensed for clarity.
Ross Andersen: To me, this is the most exciting new technology in the AI space since natural-language translation. When some of these tools first came out, I started rendering images of dreams that I had when I was a kid. I could show my kids stuff that had only previously appeared in my mind. I was wondering, since you created this technology, if you could tell us a bit about how it does what it does.
Mark Chen: There’s a long training process. You can imagine a very small child that you’re showing a lot of flash cards to, and each of these flash cards has an image and a caption on it. Maybe after seeing hundreds and millions of these, whenever there’s the word panda, it starts seeing a fuzzy animal or something that’s black and white. So it forms these associations, and then kind of builds its own kind of language for basically representing language and images, and then is able to translate that into images.
Andersen: How many images is DALL-E 2 trained on?
Chen: Several hundred millions of images. And this is a combination of stuff that we’ve licensed from partners and also stuff that’s publicly available.
Andersen: And how were all those images tagged?
Chen: A lot of natural images on the web have captions associated with them. A lot of the partners that we work with, they also provide data with annotations describing what’s in the image.
Andersen: You can do really complex prompts that generate really complex scenes. How is the thing creating a whole scene; how does it know how to distribute objects within the visual field?
Chen: These systems, when you train them, even on individual objects—it knows what a tree is; it knows what a dog is—it’s able to combine things in ways that it hasn’t seen in the training set before. So if you ask for a dog wearing a suit behind a tree or something, it can synthesize all these things together. And I think that’s part of the magic of AI, that you can generalize beyond what you trained it on.
Andersen: There’s also an art to prompt writing. As a writer, I think quite a bit about crafting sequences of words that will conjure vivid images in the mind of a reader. And in this case, when you play with this tool, the reader’s imagination has the entire digital library of humankind at its disposal. How has the way you thought about prompting changed from DALL-E 1 to DALL-E 2?
Chen: Even up to DALL-E 2, a lot of the ways people induced image generation was with short, one-sentence descriptions. But people are now adding very specific details, even the textures they want. And it turns out the model can kind of pick up on all of these things and make very subtle adjustments. It’s really about personalization—all of these adjectives that you’re adding help you basically personalize the output to what you want.
Andersen: There are a lot of contemporary artists that have been upset by this technology. When I was messing around generating my dreams, there’s a Swedish contemporary artist named Simon Stålenhag who has a style that I love, and so I slapped his name on the end of it. And indeed, it just transformed the whole thing into this beautiful Simon Stålenhag–style image. And I did feel a pang of guilt about that, like I almost wish that it was a Spotify model with royalties. But then there’s another way of looking at that, which is just, too bad—the entire history of art is about mimicking the style of masters and remixing preexisting creative styles. I know you guys are getting a lot of blowback about this. Where do you think that’s going?
Chen: Our goal isn’t to go and stiff artists or anything like that. Throughout the whole release process, we’ve wanted to be very conscientious and work with the artists, have them tell us what it is they want out of this and how can we make this safer. We want to make sure we continue to work with artists and have them provide feedback. There’s a lot of solutions that are being floated around in this space, like potentially disabling the ability to generate in a particular style. But there’s also this element of inspiration that you get, like people learn from imitation of masters.
Andersen: Neil Postman has a line that I love, where he says that instead of thinking of technological change as additive or subtractive, think about it as ecological, as changing the systems in which people operate. And in this case, those people are artists. Because you are in dialogue with artists, what are you seeing in terms of the changes? What does the creative space look like five, 10 years from now in the wake of these tools?
Chen: The amazing thing with DALL-E is we’ve found that artists are better at using these tools than the general population. We’ve seen some of the best artwork coming out of these systems basically produced by artists. The reason we built this tool is to really democratize image generation for a bunch of people who wouldn’t necessarily classify themselves as artists. With AI, you always worry about job loss and displacement, and we don’t want to kind of ignore these possibilities either. But we do think it’s a tool that allows people to be creative, and we’ve seen, so far, artists are more creative with it than regular users. And there’s a lot of technologies like this—smartphone cameras haven’t replaced photographers.
Andersen: As transformative as DALL-E is, it’s not the only show at OpenAI. In recent weeks, we’ve seen ChatGPT really take the world by storm with text-to-text prompts. I was wondering if you could say a little bit about how the evolution of those two products has made you think about the difference in textual and image creativity? And how can you use these tools together?
Chen: With DALL-E, you can get a large grid of samples and very easily pick out the one you like. With text, you don’t necessarily have that luxury, so in some sense the bar for text is a little bit higher. I do see a lot of room for these kinds of models to be used together in the future. Maybe you have a conversational interface for generating images.
Andersen: I’m interested in whether we’re ever going to get to something like an artificial general intelligence, something that can operate in many different domains instead of being really specific to one domain, like a chess-playing AI. From your perspective, is this an incremental step toward that? Or does this feel like a leap forward to you?
Chen: One thing that’s always differentiated OpenAI is that we want to build artificial general intelligence. We don’t care necessarily about too many of these narrow domains. A lot of the reason DALL-E plays into this is we wanted a way to see how our models are viewing the world. Are they seeing the world in the same way that we would describe it? We provided this text interface so we can see what the model is imagining and make sure the model is calibrated to the way we perceive the world.