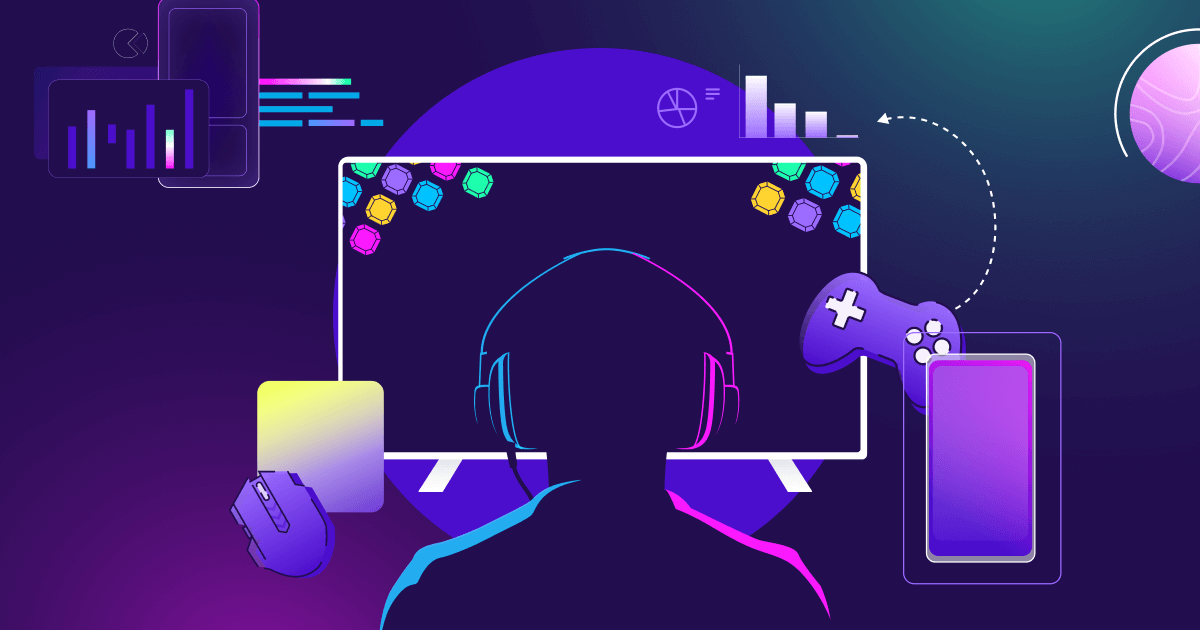
Data-driven attribution
Data-driven attribution is a marketing measurement model that assigns credit to each touchpoint along the user journey, based on how people interact with it before converting. This gives you an accurate picture of what truly drives results.
What is data-driven attribution?
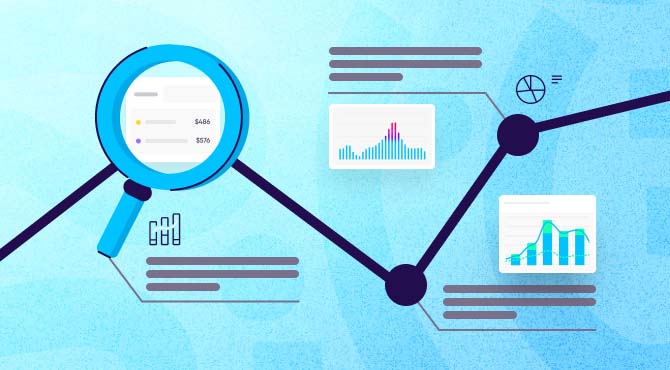
Data-driven attribution is a smart way to figure out the impact of different marketing touchpoints along the user journey.
Unlike traditional attribution models that stick to rigid rules, like giving credit only to the first or last interaction, data-driven attribution looks at the big picture. It considers every interaction a user has with your brand, both online and offline, to understand how these touchpoints work together to lead to a conversion (such as a purchase or sign-up).
This approach is much more holistic, as it dives deep into the data to uncover the true value of each touchpoint, rather than just following a set formula. As a result, you enjoy a clearer, more accurate view of complex user behavior and what’s really driving your marketing success, helping you make better, data-backed decisions.
How does data-driven attribution work?
Data-driven attribution uses machine learning and advanced algorithms to analyze every touchpoint in a user’s journey, determining how each contributes to a conversion.
A key part of data-driven attribution is using probabilistic models like the Markov chain. This model helps predict the likelihood of a user converting after interacting with various touchpoints. It analyzes the order of these interactions to identify which touchpoints are most likely to lead to a conversion and assigns credit accordingly.
Machine learning further enhances this process by continuously learning from data, improving the accuracy of credit assignment as user behavior and marketing strategies evolve.
Let’s take Google Analytics 4 as an example. GA4 uses machine learning to create a tailored data-driven attribution model that reflects the true influence of each touchpoint, with insights constantly updated to stay relevant.
Benefits of data-driven attribution
Let’s dive into they key benefits of data-driven attribution and their impact on your marketing strategy:
1 — Visualizes marketing efforts across multiple channels
Data-driven attribution offers a clear view of how various marketing channels work together along the user journey. It might show you that a user often starts with a social media ad, follows up with an email, and finishes with a Google search before converting. This interconnected view lets you see which channels pull the most weight and how they complement each other.
2 — Optimizes based on historical data
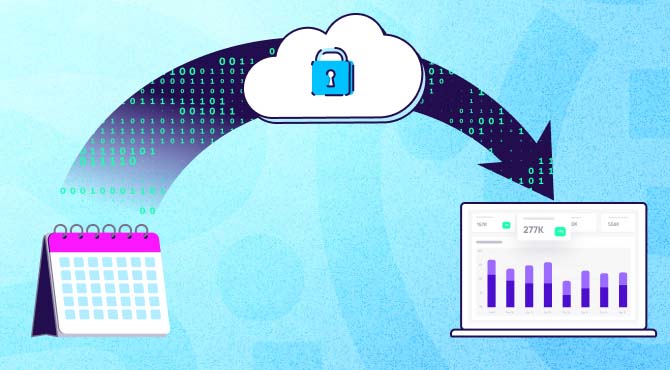
With data-driven attribution, you can use historical data to pinpoint which touchpoints and channels have delivered the best results over time. For example, you might find that combining paid search with organic content regularly leads to high-value conversions. Armed with this knowledge, you can tweak future campaigns to mirror these successful patterns, ensuring a more efficient use of your marketing budget.
3 — Helps prove marketing ROI
One of the biggest challenges in marketing is proving the return on investment (ROI) of your efforts. Data-driven attribution gives you a more accurate way to measure the impact of each marketing touchpoint, allowing you to show how your marketing activities drive revenue.
Suppose you’ve been investing heavily in display ads but haven’t seen a clear connection to conversions. Data-driven attribution might reveal that these ads play a critical role in the early stages of the user journey, justifying continued investment. This level of insight can help you defend and optimize your marketing spend.
4 — Provides valuable insights into user behavior
Data-driven attribution does more than just show you which channels are performing — it gets to the heart of how and why customers are engaging with them. This kind of insight is gold when it comes to crafting effective marketing strategies. For example, if you discover that users who watch your video content early on are more likely to convert later, you can adjust your marketing messages and tactics to better connect with their needs.
5 — Directs your ad spend and budgets more effectively

By showing you which touchpoints are most effective in driving conversions, data-driven attribution helps you allocate your ad spend and budgets more wisely.
Let’s say your model identifies that certain touchpoints, like retargeting ads, are crucial in closing sales. With this information, you can confidently increase spending on these ads while reducing budgets for less impactful channels. This ensures every dollar you spend is working hard to drive the best possible outcomes.
How does data-driven attribution compare to other attribution models?
When comparing data-driven attribution to traditional rules-based models, it’s crucial to see how each one works and where data-driven attribution stands out. Here’s how data-driven attribution stacks up against these marketing attribution models:
First-touch attribution
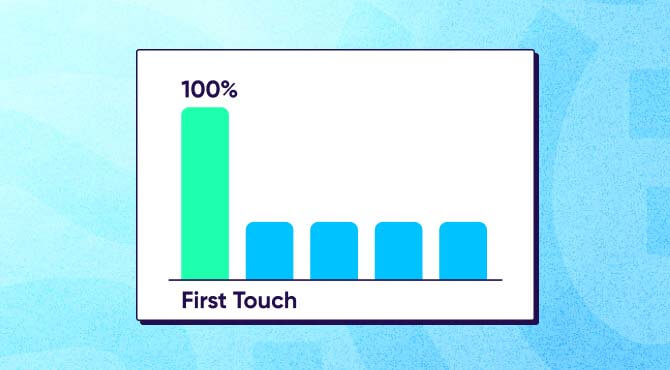
How it works: Gives all the credit for a conversion to the very first touchpoint the customer interacted with.
While first-touch (or first-click) attribution is handy for spotting which channels spark initial interest, it ignores the influence of later touchpoints that may have sealed the deal. Data-driven attribution, however, spreads the credit across the entire user journey, recognizing the real impact of each touchpoint.
Last-touch attribution
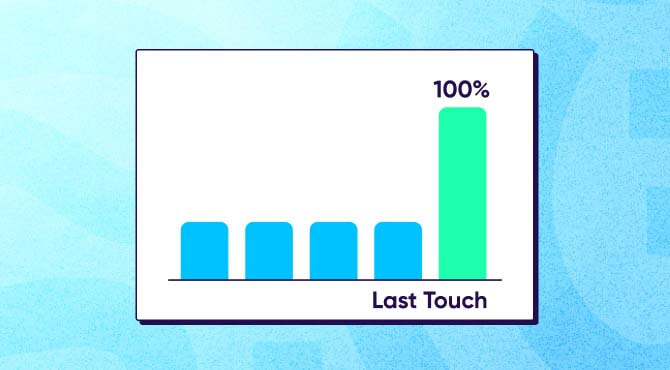
How it works: Attributes 100% of the conversion credit to the last touchpoint the user interacted with before converting.
Last-touch (or last-click) attribution assumes the final interaction is solely responsible for the conversion, ignoring the contributions of earlier touchpoints. That’s a very limited approach. In comparison, data-driven attribution offers a more nuanced view, showing how each touchpoint influenced the final decision and ensuring credit is given where it’s due.
Time decay attribution
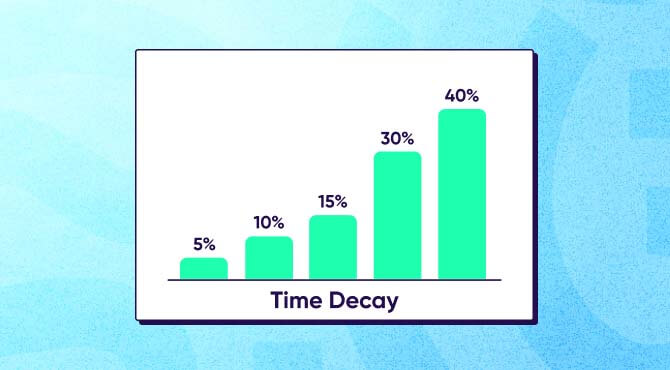
How it works: Gives more credit to touchpoints that occurred closer in time to the conversion. The further back a touchpoint is, the less credit it receives.
Time decay attribution recognizes that touchpoints closer to the conversion might have a stronger influence, but it still follows a set rule. Data-driven attribution, however, skips the assumptions, using real data to assess each touchpoint’s true contribution, no matter when it happened.
Linear attribution
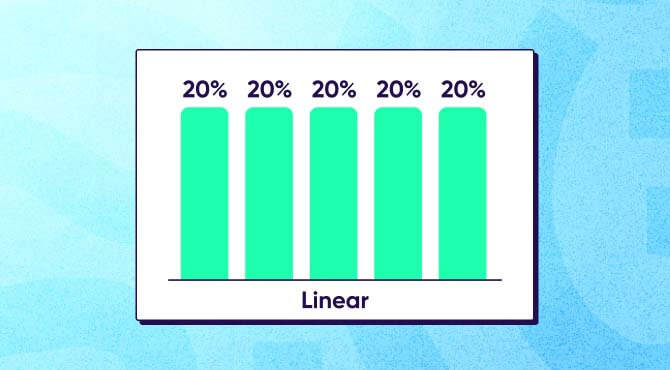
How it works: Distributes conversion credit evenly across all touchpoints.
Linear attribution treats all touchpoints equally — which might sound fair, but can oversimplify the user journey. Data-driven attribution improves on this by analyzing the actual impact of each interaction, allocating credit based on how much each touchpoint genuinely contributed to the conversion.
Position-based attribution

How it works: Gives most credit to the first and last touchpoints (and sometimes a middle one), with the remainder spread equally across the other interactions.
Position-based attribution tries to balance the importance of the first and last interactions while giving some credit to the middle ones. The U-shaped model gives the majority of credit to the first and last touchpoints, splitting the rest equally among those in between. The W-shaped model has an additional “spike” in the middle, recognizing the importance of an intermediate touchpoint.
While adding some nuance, these models still follow an arbitrary distribution of credit. Data-driven attribution doesn’t rely on fixed rules — it looks at how users engage with each touchpoint throughout their journey, reflecting the actual contribution of each touchpoint based on real data.
Limitations of data-driven attribution
While data-driven attribution offers a lot of benefits, it does come with a few challenges that are worth keeping in mind:
- Requires high volumes of data: For data-driven attribution to work its magic, it needs a lot of data to analyze. Without sufficient data, the model’s insights may be less reliable, particularly for smaller campaigns or brands with limited user interactions.
- Complex to implement and optimize: Setting up and optimizing a data-driven attribution model isn’t exactly a plug-and-play situation. It can get pretty technical, so you might need some expert help or specialized tools to get it right and keep it running smoothly.
- Data privacy regulations: With all the data privacy rules out there, such as GDPR, collecting and using user data for attribution is tricky. You’ll need to make sure you’re staying compliant, but this can sometimes limit the data you have available, which might affect the accuracy of your attribution model.
- Credit is not assigned to non-ad touchpoints: Data-driven attribution usually focuses on advertising touchpoints, which means it might miss out on other important interactions. Think: word-of-mouth referrals or in-store experiences.
- Hard to see how attribution is modeled: The algorithms behind data-driven attribution can be pretty complex, making it tough to fully understand how credit is being distributed. This “black box” nature can make it challenging to explain or justify the results to stakeholders.
How to implement data-driven attribution

Finally to the fun bit!
Let’s decode how you can implement data-driven attribution.
1 — Set your attribution goals
First up, figure out what you want to achieve with data-driven attribution. Is it to discover which marketing channels are the heavy lifters for conversions? Or maybe you want to fine-tune your budget across different touchpoints? Knowing your goals will shape how you set up and measure your attribution model.
For example, if boosting app installs is your aim, your model should spotlight the touchpoints that are the real MVPs in driving those downloads.
2 — Identify conversion paths and marketing touchpoints
Now, map out the user journey — from the first hello to the app download and beyond. Think about every interaction, from social media ads to in-app referrals. The more detailed you get, the better your model will mirror the real-world customer journey.
Keep a close eye on app store optimization (ASO) and how users bounce between your ads and the app store. Knowing these key touchpoints will help you see where potential users are engaging with your app marketing and what’s pushing them to convert.
3 — Start collecting data
The next step is to set up tracking across all relevant channels. For mobile apps, this usually means integrating an SDK (software development kit) to measure installs, in-app behavior, and more. Gather data from ad networks, social media, app stores, and don’t forget those crucial in-app events like first purchases or level completions.
And of course, keep it legal! Make sure you’re in line with privacy regulations like GDPR and CCPA when handling user data.
4 — Analyze the data for insights
With data in hand, it’s time to dig in. Look at which touchpoints are driving the best results — like app installs or repeat purchases. You might spot trends, like users who see both a Facebook ad and an in-app notification being more likely to buy.
Advanced analytics tools can help you identify these patterns and understand how different touchpoints work together to boost user acquisition and retention.
5 — Optimize your campaigns
Based on the insights from your data, refine your app marketing strategy. This could mean reallocating budget towards channels that bring in high-quality installs, or personalizing push notifications based on ad interactions.
For instance, if users who install your app after watching a video ad are more engaged, then you might want to double down on video content in your campaigns. The goal is to make sure every marketing dollar is working hard to drive user acquisition and retention.
6 — Ongoing training of the model
The only constant is change, and that goes for your attribution model too. As user behavior evolves, keep gathering new data — especially after big app updates or shifts in your marketing strategy — and retrain your model to stay on point.
Adjust the weight of certain touchpoints or explore new channels that are trending. Regularly fine-tuning your model ensures it stays in sync with the ever-changing mobile app marketing trends, keeping you ahead of the game.
Key takeaways
- Unlike traditional attribution methods, data-driven attribution looks at the entire user journey, considering every touchpoint — online and offline. This gives you a clearer, more complete picture of what really drives conversions.
- Data-driven attribution uses machine learning and probabilistic models to continuously learn from data, improving how it assigns credit to each touchpoint based on its actual impact on conversions.
- Data-driven attribution offers a more accurate way to measure and prove the ROI of your marketing efforts. By showing exactly how each touchpoint contributes to revenue, it helps you justify and optimize your marketing spend.
- Getting started with data-driven attribution isn’t without its challenges. It’s a complex model that requires a lot of data, technical know-how for setup and fine-tuning, and careful attention to data privacy regulations.
- The key to success with data-driven attribution is keeping the model up to date. As new data comes in and user behaviors shift, continuous training is essential to ensure the insights stay relevant and accurate.