Effect of Obstacle Type and Cognitive Task on Situation Awareness and Takeover Performance in Conditionally Automated Driving
Effet du type d'obstacle et de la tâche cognitive sur la conscience de la situation et la performance de reprise de contrôle en conduite conditionnellement automatisée
DOI: https://doi.org/10.1145/3583961.3583966
IHM '23: IHM '23: Proceedings of the 34th Conference on l'Interaction Humain-Machine, TROYES, France, April 2023
In conditionally automated driving, several factors can affect the driver's situation awareness and ability to take over control. To better understand the influence of some of these factors, 88 participants spent 20 minutes in a conditionally automated driving simulator. They had to react to four obstacles that varied in danger and movement. Half of the participants were required to engage in a verbal cognitive non-driving-related task. Situation awareness, takeover performance and physiological responses were measured for each situation. The results suggest that obstacle movement influences obstacle danger perception, situation awareness, and response time, while the latter is also influenced by obstacle danger. The cognitive verbal task also had an effect on the takeover response time. These results imply that the driver's cognitive state and the driving situation (e.g. the movement/danger of entities present around the vehicle) must be considered when conveying information to drivers through in-vehicle interfaces.
En conduite automatisée, plusieurs facteurs peuvent affecter la conscience de la situation et la reprise de contrôle. Pour mieux comprendre cela, 88 participants ont passé 20 minutes dans un simulateur de conduite conditionnellement automatisée. Ils devaient réagir à quatre obstacles dont le danger et le mouvement variaient. La moitié des participants devaient s'engager dans une tâche cognitive verbale non liée à la conduite. La conscience de la situation, la performance et les réponses physiologiques ont été mesurées. Les résultats suggèrent que le mouvement de l'obstacle influence la perception du danger, la conscience de la situation et le temps de réaction, ce dernier étant également influencé par le danger de l'obstacle. La tâche cognitive verbale a également un effet sur le temps de réaction. Ainsi, l’état cognitif du conducteur et la situation de conduite (mouvement/danger des entités autour du véhicule) devraient être considérés lors de la transmission d'informations via les interfaces homme-véhicule.
ACM Reference Format:
Quentin Meteier, Marine Capallera, Emmanuel De Salis, Leonardo Angelini, Stefano Carrino, Omar Abou Khaled, Elena Mugellini, and Andreas Sonderegger. 2023. Effect of Obstacle Type and Cognitive Task on Situation Awareness and Takeover Performance in Conditionally Automated Driving. In IHM '23: Proceedings of the 34th Conference on l'Interaction Humain-Machine (IHM '23), April 03--06, 2023, TROYES, France. ACM, New York, NY, USA 12 Pages. https://doi.org/10.1145/3583961.3583966
1 INTRODUCTION
In most cases, road accidents are caused by human errors rather than technical failures [49, 54]. This is usually due to a number of factors that can affect the driver's state, such as drowsiness, distraction or stress. These factors are well known to deteriorate driving performance, sometimes up to the point of causing an accident [15, 24, 34, 39]. To address this problem, car manufacturers have increased the level of automation in vehicles to assist drivers. The taxonomy published by the Society of Automotive Engineers (SAE) is the reference for definitions and terms related to automated driving systems [23]. According to this taxonomy, the generation of conditionally automated vehicles (SAE Level 3) is the next one that is gradually emerging on our roads. In the rest of the manuscript, the level 3 automation corresponding to the SAE taxonomy will be referred to as L3-SAE. At this level, drivers will no longer be in charge of the dynamic driving task, nor of monitoring the environment. However, they should be able to regain control if a critical situation occurs that cannot be handled by the vehicle. In such a case, the vehicle sends a takeover request (TOR) to the driver. Certain factors may affect the ability of drivers to take over control, including the psychophysiological state of the driver, but also the environmental complexity at the time of the takeover request.
The driver's state must be optimal in order to effectively regain control of the vehicle when necessary. Factors such as sleep-related fatigue or pre-driving stress can affect the driver's initial state. In addition, conditionally automated driving allows drivers to engage in a non-driving-related task (NDRT). Depending on the task being performed, drivers may take their eyes off the environment completely, thereby reducing their situation awareness (SA). Performing an NDRT could also increase drivers’ mental workload and therefore affect their performance with the automated system [53, 58]. Some studies have already suggested that engaging in an NDRT deteriorates the takeover performance in automated driving [15, 34, 39, 51, 57, 59]. A poor driver condition when entering the vehicle, combined with a highly distracting and cognitive task during automated driving, could be fatal when a takeover is needed.
In addition, depending on the state of the driving environment when the vehicle triggers a TOR, it may be longer and more difficult for the driver to understand the situation and react accordingly. Several factors such as the environmental complexity can have an influence on the driver's understanding and response time [15, 25, 44]. Indeed, a more complex driving situation could induce a greater mental workload, reduce SA and thus lead to a weakening of the takeover performance [17, 41].
In previous research, the influence of the NDRT engagement on takeover performance in automated driving has been already addressed [15, 34, 39, 51, 57, 59]. However, the impact of the driving environment on SA and takeover performance has received less attention, especially in conditionally automated driving (L3-SAE).
2 RELATED WORK
2.1 Influence of the driving environment and non-driving-related task on SA and performance
If there are still accidents on the roads, it is partly due to the fact that the driving task can be considered a complex dynamic task for humans. Indeed, a driver needs to process much information from the driving situation and make decisions to ensure acceptable driving performance. The concept of situation awareness (SA) emerged in the literature to theorize this internal process in the human brain before decision-making and performance of action when interacting with dynamic systems (e.g., a plane or a car). Endsley [17] defined SA as the perception of the elements in the environment within a volume of time and space, the comprehension of their meanings and a projection of their status in near future. According to her three-level model, SA is related to decision-making and task performance. Previous studies showed that a higher SA leads to better decisions and increased performance while interacting with an automated system [18]. In the context of driving, SA implies that the driver is able to perceive and understand what is happening in the surrounding area of the car at any time and act adequately, even while performing an NDRT. The same model also highlights several factors that can affect driver SA, such as task and environmental factors (e.g. workload, stressors, complexity) or individual factors (e.g. knowledge, experience) [17]. This means that not only the driver's workload level but also the complexity of the driving situation should have an impact on SA and potentially on driving performance. Several works have studied the influence of different aspects of the driving environment on SA and performance, both in manual and automated driving.
[25] investigated the effect of environment complexity (simple – rural vs. complex – city) with two types of hazard exposure (static vs. dynamic) on SA and driving performance. After hazard exposure, they found degradation of all SA indices in the city compared to the rural environment, which confirms the influence of environment complexity on SA as suggested by Endsley's model [17]. For other levels of SA, there was also an interaction effect with age, which can be included in individual factors impacting SA in Endsley's model. In addition, the type of hazard had an influence on the speed ratio during hazard exposure (higher speed reduction when encountering dynamic hazard). In another study, the environment complexity manipulated through the amount of information on traffic signs affected negatively driver workload and thus manual driving performance (lower speed and increase of lane departure) [28].
In automated driving, studies also found that the environmental complexity and the type of hazard exposure affect negatively SA and takeover performance. A higher traffic density seems to impair the takeover performance [15, 44], while the takeover quality improves with a rise in familiarity (i.e., repetition of situations) [44]. [11] also found an effect of hazard direction (static in front vs moving on the side), which had a significant effect on takeover performance (steering wheel grip and hazard response time) but also on SA. Drivers were quicker to grip the steering wheel for static obstacles in front. Besides, drivers had a higher SA (measured subjectively) than when faced with moving obstacles (coming from the side) than for static obstacles (in front). The opposite pattern would have been expected since the movement of obstacles would create a more variable and uncertain situation [17, 20, 48]. More recently, [1] found correlations between SA and physiological measures. Also, a negative effect of change in the physiological driver's state was found on takeover performance. Hence, it might be relevant to assess the driver's physiological state to ensure a proper takeover performance.
While environmental factors were found to affect drivers’ SA and performance, the nature of the task performed by an operator is expected to influence drivers’ SA (and thus performance) according to Endsley [17]. Previous automated driving studies showed that engaging in different NDRT impairs takeover performance. [15, 34, 39, 57]. Participants were asked to engage in the N-back or twenty questions task, interact with an entertainment console or a smartphone, watch a movie, read a book or listen to an audiobook. The driving performance was evaluated by different metrics such as the response (or reaction) time, gaze on time, hands-on-time, the standard deviation of lateral position, first braking or the steering manoeuvre. Also, Wulf et. al [56] also show a negative impact of NDRT on SA in addition to driving performance.
2.2 Measuring situation awareness, takeover performance and driver's state
2.2.1 Situation awareness. Various techniques have been suggested in the literature to infer SA such as freeze-probe techniques, real-time probe techniques, post-trial self-rating techniques, observer-rating techniques, performance measures and process indices [40]. A widely used technique is the freeze-probe one, in which the simulation is stopped at a specific moment and the operator/driver is asked what they perceived and understood from that situation. The Situation Awareness Global Assessment Technique (SAGAT) [16] is one of the most used questionnaires to measure SA after freezing the simulation. When the simulation cannot be stopped, the post-trial self-rating technique is often employed. A questionnaire is used to assess the operator's SA after completing a task and a well-known instrument to do that is the Situation Awareness Rating Technique (SART) [48]. It was originally developed for the assessment of pilots’ SA but items are generic so this questionnaire was also used in studies related to (automated) driving [27, 50]. SA assessment can also be combined with process indices measures such as verbal protocols ("think aloud" technique) [43].
2.2.2 Takeover performance. There are two different approaches to evaluate the drivers’ takeover performance at L3-SAE. Depending on the situation, the driver usually has a set of expected reactions, and any behaviour outside of this set is considered unsuitable. On the other hand, a set of performance metrics were defined and used in previous literature [52], and some of the most used ones are described below:
- Response time (RT) [19] is the difference in the time between the takeover request and the first action of the driver that turns the automation off, usually either braking or turning the steering wheel. RT should not be used alone, as a low RT could sometimes indicate a precipitated reaction, which is not ideal. The term "reaction time" is also used in the literature, depending on what is measured during the study (measurement of a reflex or a more complex behaviour).
- Maximum Steering Wheel Angle (MaxSWA) [4] is the maximum angle reached by the steering wheel during the whole takeover process, which is from the actual takeover of the driver until the conditional automation is resumed. A lower MaxSWA often indicates a better takeover quality.
- Crash [38] is a simple Boolean metric, which indicates that the car has collided with a static obstacle or other physical entity during the takeover process. A crash brings the vehicle to a complete stop and causes damage, while a simple collision does not necessarily stop the vehicle.
2.2.3 Driver's state. Reacting to a takeover request and handling a dangerous situation in a short period of time can be stressful. The autonomic nervous system is known to be affected by stress [55]. Ultra-short-term indicators (less than five minutes) of heart rate variability can quantify the activation of the autonomic nervous system. According to [45], the heart rate can be calculated from 10 seconds of an electrocardiogram, while 30 to 60 seconds could be enough to get a reliable measure of other temporal indices of cardiac activity in a very short period of time. In addition, the electrodermal activity (EDA) of the body is also controlled by the autonomic nervous system [13]. The response to a stressful stimulus can hence be characterised by the occurrence of a Skin Conductance Response (SCR). It is defined as a quick increase of skin conductance, usually within 1 to 5 seconds [3]. The intensity of the physiological response to a stressful stimulus can be characterised by several skin conductance indicators such as the peak amplitude, the rise time (to reach peak amplitude from onset) and the half-recovery time of SCR (i.e., the time it takes for SCR to decrease to half amplitude) [3, 13]. Some of these measures might be giving relevant information on the driver's physiological response when reacting to stressful takeover situations.
3 CURRENT STUDY
Previous studies investigated the impact of environmental factors on drivers’ SA and performance, in both manual and automated driving [1, 11, 15, 25, 28, 44]. The environment complexity was often manipulated through the type of environment [25] or the traffic (signs) density [15, 28, 44], while the type of hazard exposure was also tested [11]. In addition, driver engagement in an NDRT also showed degradations in SA and driver performance [15, 34, 57]. However, its interaction effect with the complexity of the environment and the dangerous nature of the hazard causing the TOR has not been investigated.
Therefore, the primary objective of this study is to examine the influence of an environmental factor that has been less investigated in previous research, namely the type of hazard exposure causing a takeover request. Its effect on the driver's SA, takeover performance, and physiological response in conditionally automated driving (L3-SAE) is tested. However, the manipulation of the type of hazard exposure was done differently than in previous research, with the type of obstacle leading to TORs varying in movement but also in danger (i.e., the potential for causing damage). A second objective is to confirm the negative effect of the NDRT on SA and takeover performance and observe its interaction effect with the type of hazard exposure on the same measures.
To answer these research questions, half of the drivers engaged in a verbal cognitive NDRT throughout the experiment. All participants faced four critical situations where the car asked them to take over control. SA, takeover performance, and physiological response were measured. On the basis of the literature review, we formulate the following hypotheses:
- (H1): The movement of the obstacle should have a negative effect on SA. It should not change anything at the stage of perception of SA (level 1 according to Endsley's model [17]). However, the uncertainty of the obstacle's movement should make the situation more unstable and variable, leading to a decrease in SA at the comprehension (level 2) and projection (level 3) stages of SA. Therefore, we assume that drivers should have a higher SA when the obstacle is static than when it is moving. [11, 17, 48]. Consequently, the physiological response should be greater, linked to the stress generated by the uncertainty of the obstacle's movement or to greater driver's attention [20, 25]. A significant difference should be found in the takeover performance based on the results obtained in a previous study (reduction of speed when facing moving obstacles) [25]. Drivers could delay the decision to take over control of the vehicle if faced with a moving obstacle, because they might spend more time at levels 2 (comprehension) and 3 (projection) of the SA process once a TOR is triggered.
- (H2): If we look at the three stages of the SA process, the danger of the obstacle should not particularly impact the driver's perception (level 1) and comprehension (level 2) of the driving situation. On the other hand, it could impact his/her projection (level 3) before taking the decision to regain control or not, as a dangerous obstacle could do more damage. However, there is no evidence in the literature suggesting that the danger of the obstacle has an impact on the driver's SA and takeover performance. Thus, we hypothesise that it will not have an impact on SA and takeover performance in our study either. Furthermore, a dangerous obstacle should induce more stress in the driver and thus result in a significant increase in heart rate and skin conductance [9, 12, 55].
- (H3): Driver engagement in a verbal cognitive NDRT is expected to increase drivers’ mental workload prior to regaining control [32, 33, 42] and thus have a negative effect on SA and takeover performance [15, 34, 39, 56].
4 METHOD
4.1 Participants and Experimental Design
88 young drivers (24.19 ± 6.05) were recruited for this study. 40 considered themselves to be male, and 48 considered themselves to be female. All participants were required to have a valid driving license and be in good general health. Participants who were university students received course credit for their participation. All research and measurements followed the principles of the Helsinki agreement and written consent was obtained from all participants. On average, the participants held their driving license for around 5 years (4.68 ± 6.12) and reported driving around 3800 kilometres per year (3772.92 ± 6468.44). 72 of them did not have any accidents in the three years preceding the experiment.
A 2 x 2 x 2 mixed experimental design was adopted for this experiment. The type of obstacle triggering a TOR, namely movement (moving vs. static) and danger (danger vs. non-dangerous), was manipulated as two within-subject factors, while the type of NDRT performed by the driver (observing the vehicle environment vs. verbally counting backwards) was manipulated as a between-subject factor.
The obstacles that elicited the TORs were a deer, a traffic cone, a frog, and a can. The four obstacles are illustrated in Figure 1. These obstacles were chosen because it is likely that each of them could be encountered in a rural environment such as the one used in the study. Moreover, the modelling effort remained acceptable and thus allowed the desired experimental manipulation to be carried out.
Firstly, the obstacles vary in terms of movement. The deer and the frog are mobile obstacles as they move and cross the road when the car approaches, from right to left. In contrast, the traffic cone and the can are static obstacles located in the middle of the road. This distinction between static and mobile entities has been already done in a taxonomy classifying the limitations of automated vehicles (obstacles vs. external human factor) [7].
Secondly, the obstacles vary in terms of danger. This is reflected in the potential of the obstacle to cause damage to the car and driver, due to its size and/or weight. The deer and the traffic cone were considered dangerous, while the frog and the can were considered non-dangerous. The order of appearance of the obstacles was controlled between participants according to a Latin Square design [26].

4.2 Material and instruments
The experiment was conducted in a fixed-base simulator, as shown in Figure 2. It consists of two adjacent car seats, and a Logitech G27 steering wheel with accelerator and brake pedals. The orientation and position of the seats could be adjusted by the driver. This unit is installed in front of a large screen onto which the driving simulation was projected from behind using a projector (model Epsilon EH-TW3200). Two speakers are placed behind the seats to immerse the driver in the simulated environment. The whole system is located in a cabin with low ambient lighting.
The software used for the driving simulation is GENIVI. The driving scenario is a replica of Yosemite National Park (USA). A continuous 20-minute drive with conditionally automated driving (L3-SAE) features was implemented. Drivers could take over control of the car by turning the wheel, braking, or pressing a button on the steering wheel. A TOR was requested by the car to the driver by means of a red icon on the dashboard and a sound signal (see Figure 2). Obstacles causing the TORs were also implemented in the driving scenario (see Figure 1). The nature of the obstacles was not mentioned or shown to the participants before the experiment. For each obstacle, the initial time to collision was 7 seconds, which is generally chosen as the average time sufficient for the driver to react and regain control [52]. The car was driving at 53 km/h and the initial distance between the car and the obstacle at the time of the TOR was therefore about 100 meters.
Physiological signals (ECG and EDA) of the drivers were collected continuously during the experiment using the Biopac MP36 hardware. A sampling rate of 1000 Hz was used for signal acquisition. A digital low-pass filter with a frequency of 66.5 Hz and a Q factor of 0.5 was applied to both signals. In addition, a gain of 2000 was added for the EDA signal. Two sets of SS57LA and SS2LB probes (Biopac) with disposable Ag/AgCl pre-gelled electrodes (EL507 and EL503, Biopac) were used to record the EDA and ECG of the participants, respectively.

4.3 Measures
To check whether the type of obstacle was correctly manipulated experimentally, participants were asked to rate the realism (i.e., "How realistic did the obstacle seem to you?") and perceived danger (i.e., "How dangerous did the obstacle seem to you?") for each obstacle that elicited a TOR. This assessment was made on a 7-point Likert scale, ranging from "not dangerous/realistic" to "very dangerous/realistic". Engagement in the NDRT was monitored by the number of digits spoken per minute. The number of times the experimenter asked the participant to resume counting was also recorded.
Physiological signals were also used to control the manipulation of the driver's cognitive state before the TORs through engagement in a NDRT. In particular, the average heart rate calculated over a two-minute period prior to the TOR and corrected with the baseline value was used [10, 31, 32]. The experimental manipulation was also controlled through the subjective assessments of the NASA Task Load Index (NASA-TLX) questionnaire [22], reported at the end of the experiment. The aggregated score from the six components was used, also known as Raw TLX (RTLX) [21]. No weighting procedure was applied since it was only a control measure and mixed results were found in the literature [21].
SA was assessed for all four situations. As the simulation could not be stopped due to the continuous measurement of physiological signals throughout the experiment, freeze-probe assessment techniques could not be used in this study. Therefore, the SART was chosen as the self-evaluation technique after the experiment [48]. This questionnaire was selected because it is quick, easy to implement, and widely used. A picture of the situation was proposed to drivers in addition to the questionnaire when assessing their SA for each obstacle [40].
As the SART only provides a subjective measure of SA after the experiment, an alternative measure of participants’ SA was collected, considered to be a performance measure according to [40]. Due to the limited time available after the TOR, it was not possible to use a verbal protocol. Therefore, participants were asked to press a button on the steering wheel from the moment they understood the driving situation, after the TOR. The time each participant took to understand the situation, between the TOR and pressing the button, was measured for all situations and all participants. This measurement is referred to as the Time to Understand the Situation (TUS) in the following.
Regarding the takeover of the vehicle, whether the participant regained control of the car or not was extracted from the raw driving data. As indicators of takeover performance, the RT and the MaxSWA during the maneuver were chosen as they are often used in the literature [4, 19, 52]. Their definition follows the one given in section 2.2.2. The calculation of these measures for each situation and each participant was automated thanks to a code implemented in Python.
To measure the physiological responses of the drivers during the takeover situation, an automated calculation of physiological features was performed using the Neurokit library in Python [30]. A time window ranging from 1 second before to 8 seconds after the TOR was used to calculate the physiological measurements of the drivers during each situation (time window less than 10 seconds imposed by the Neurokit library). From the EDA signal, two indicators characterising a stimulus-associated SCR were calculated: the maximum amplitude and the rise time (to reach the maximum amplitude from the onset) [3]. As an indicator of cardiac activity during the same period of time, the average heart rate during the situation was used. These measures were selected as they are sensitive to stress [9, 12, 55], which could occur during takeover situations.
4.4 Experimental procedure
To begin with, the participants answered the first part of the questionnaire with socio-demographic questions as well as questions about their driving habits. Then, the experimenter set up the electrodes for recording physiological signals. The experiment was divided into three phases, each using the same driving environment described above.
The first phase (Baseline) of the experiment was used to measure physiological indicators for each participant at rest. For five minutes, participants were asked to observe the environment of the car while it was driving in conditional automation (L3-SAE). The participants were informed that no TOR could occur during this phase.
The second phase (Training) of the experiment was the training session. During this five-minute phase, participants were familiarised with the driving simulator and the takeover request process. Participants were reminded that they were driving a L3-SAE vehicle. The meaning of the icons indicating the autopilot status on the dashboard was explained by the experimenter. Each driver was given three "false" TORs (i.e., no obstacles in the lane) to familiarise themselves with the process. After that, the experimenter ensured that the participants understood the process and the participants had to drive manually until the end of the five minutes.
The third phase (Driving) was the main driving session. Participants were in the driving simulator for 20 minutes while the car was driving in conditional automation. Before starting, participants were instructed to take over control of the car after a TOR only when they considered that the obstacle could cause damage to them and the car. They were also asked to press a button on the steering wheel once they had seen the obstacle and understood the situation after the TOR. In total, each participant had to react to the four situations involving the different obstacles presented earlier. If the drivers took over control, they were instructed to resume the autopilot by repositioning the car on the right lane and pressing a steering wheel button once the situation was not critical anymore (according to them). Each TOR was randomly triggered between 1.5 minutes and 4 minutes after the previous situation. Half of the participants were also asked to perform the cognitive NDRT which was counting backwards from 3645, from 2 to 2. At the end of the 20 minutes of driving, participants were asked to stop the car and leave the simulator. The electrodes were removed, the participants completed the last part of the questionnaire and were finally dismissed.
4.5 Statistical analysis
Some data could not be used or had to be excluded from the statistical analysis, except for danger and realism measures. The data from seven participants were excluded because a problem occurred with at least one obstacle triggered by the experimenter (three with the traffic cone, three with the frog and one with the can). The TOR was triggered but no obstacle appeared in the environment. Also, one participant experienced motion sickness so data were not considered in the analysis.
Concerning the physiological data, three participants were excluded because of synchronization problems between timestamps and collected data. Also, the physiological features indicators could not be calculated for some of the participants primarily due to noise in the signal during takeover situations. The data of 34 participants was considered for the statistical analysis of the SCR amplitude and rise time, while the data of 75 participants was used for the analysis of heart rate during the takeover situations.
For measures of TUS, RT and MaxSWA, thresholds for data exclusion have been used. According to the study of Bazilinskyy [2], the average RT to an intense audio-visual stimulus without onset asynchrony is 280 milliseconds on average. Participants who pressed the button or took over control in this short period of time may have only reacted to the stimulus and did not take time to understand the situation. Hence, they were excluded from the analysis. Also, participants who pressed the button or took over control after more than 7 seconds (i.e., time to collision after TOR) were also excluded, because it was already too late to understand the situation and/or take over control of the vehicle. For MaxSWA measures, values higher than 180 degrees (more than half a turn of the wheel) were removed from the analysis, since they were outliers. Indeed, participants were not supposed to do more than half a turn with the wheel.
A mixed repeated measures analysis of variance (ANOVA) was done to investigate the effect of movement and danger of obstacles, NDRT, and the interaction effects between these factors on all dependent variables. A t-test was done to control for the inducement of workload through NASA-TLX measures. Figures are proposed to interpret the interaction effects when they are significant.
5 RESULTS
5.1 Manipulation check
5.1.1 Subjective ratings of danger and realism of obstacles. Data analysis shows a significant effect of the danger (F(1, 79) = 247.07, p < .001, η2 = .36) and the movement (F(1, 79) = 51.07, p < .001, η2 = .07) of obstacles on subjective ratings of danger reported by participants. The obstacles considered dangerous in the experimental design (the deer and the cone) were rated as more dangerous (M = 4.89, SE = 0.13) than obstacles considered non-dangerous (M = 2.48, SE = 0.13). Besides, moving obstacles (the deer and the frog) were rated as more dangerous (M = 4.21, SE = 0.13) than static obstacles (M = 3.15, SE = 0.13). The interaction effect of danger and movement is also significant (F(1, 79) = 7.78, p < .01, η2 = .01), which is shown in Figure 3. A higher difference can be observed in ratings between moving and static obstacles when they are considered dangerous than when they are considered non-dangerous. The effect of NDRT and other interaction effects did not reach significance level (p > .05).

Data analysis also shows a significant effect of the danger (F(1, 79) = 9.23, p < .01, η2 = .02) and the movement (F(1, 79) = 10.97, p < .001, η2 = .02) of obstacles on subjective ratings of realism reported by participants. The obstacles considered as dangerous in the experimental design (the deer and the cone) were rated as more realistic (M = 5.29, SE = 0.14) than obstacles considered as not dangerous (M = 4.85, SE = 0.14). Besides, moving obstacles (the deer and the frog) were rated as less realistic (M = 4.83, SE = 0.14) than static obstacles (M = 5.30, SE = 0.14), suggesting that the animation of animal movement could be improved. Besides, the effect of NDRT and all interaction effects on realism did not reach the significance level (p > .05).
5.1.2 Engagement in NDRT. Participants who were asked to engage in the verbal NDRT counted backwards, on average, to the number 2740 (M = 2740.03, SD = 311.28), making an average of 452 calculations throughout the driving session (M = 452.49, SD = 155.64). It corresponds to 22.6 numbers orally spoken per minute, so approximately one number every three seconds. The experimenters asked participants to resume counting on average twice (M = 2.58, SD = 1.71).
5.1.3 Inducement of mental workload through NDRT. Participants who engaged in the NDRT reported to have a higher level of MWL (M = 4.66, SD = .89) compared to the control group (M = 3.90, SD = 1.44; t(77) = -2.82, p < .01, d = -0.63), regarding the aggregate score of the NASA-TLX questionnaire (RTLX). An effect of the NDRT was also found on the mean heart rate of participants (corrected with baseline) (F(1, 75) = 59.21, p < .001, η2 = .35). The mean heart rate of participants who engaged in the NDRT increased compared to baseline (M = 6.75, SE = .72), while it decreased for participants in the control group (M = -1.10, SE = .72).
5.2 Situation Awareness
5.2.1 SART. The data analysis shows a significant effect of the movement of obstacles on drivers’ subjective SA (F(1, 78) = 44.96, p < .001, η2 = .10). Participants reported to have a lower SA when facing the moving obstacles (M = 18.23, SE = .61) compared to facing the static ones (M = 22.42, SE = .61). Besides, there is also an interaction effect of movement and danger on the drivers’ subjective SA (F(1, 78) = 5.03, p < .05, η2 = .005). This interaction effect is shown in Figure 4. When facing static obstacles, drivers’ SA was higher for the non-dangerous obstacle (can) than the dangerous one (traffic cone). However, drivers’ SA was higher for the dangerous obstacle (deer) than the non-dangerous one (frog) when facing moving obstacles. Besides, there is no significant effect of danger and NDRT alone, as well as no interaction effects (p > .05).
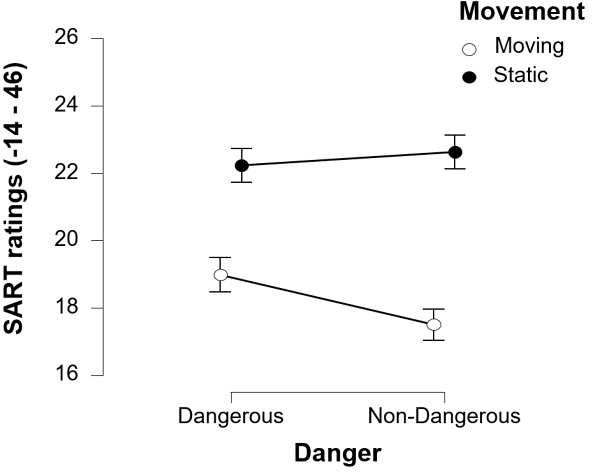
5.2.2 Time to understand the situation (TUS). The data analysis shows a significant effect of the movement (F(1, 61) = 13.59, p < .001, η2 = .03) and danger of obstacles (F(1, 61) = 12.13, p < .001, η2 = .03) on TUS. Participants took more time to understand the situation and press the button on the wheel when they faced static obstacles (M = 2.19 s, SE = .09 s) compared to moving ones (M = 1.86 s, SE = .09). They also took more time to understand the situation and press the button on the wheel when they faced dangerous obstacles (M = 2.18 s, SE = .10 s) compared to non-dangerous ones (M = 1.87 s, SE = .10). Besides, no significant effect of NDRT was found, as well as no interaction effects (p > .05).
5.3 Takeover performance
Table 1 shows the number of participants who took over control of the car in the takeover situations involving each obstacle. Besides, the statistical analysis revealed a significant effect of obstacles’ movement on RT (F(1, 34) = 14.53, p < .001, η2 = .07). Participants took more time to take over when the obstacle triggering the TOR was moving (M = 3.59 s, SE = .15 s) than when it was static (M = 2.97 s, SE = .12 s). Also, the effect of obstacles’ danger is significant on RT (F(1, 34) = 6.42, p < .05, η2 = .02). Participants took less time to take over when the obstacle was considered dangerous (M = 3.10 s, SE = .14 s) than when it was considered non-dangerous (M = 3.46 s, SE = .12 s). The effect of NDRT on RT is also significant (F(1, 34) = 8.11, p < .01, η2 = .07). Participants who engaged in the verbal cognitive NDRT took more time to take over control (M = 3.59 s, SE = .15 s) than those in the control group (M = 2.97 s, SE = .16 s). No interaction effects were found on RT (p > .05).
Deer | Traffic Cone | Frog | Can | |
Takeover | 86 | 87 | 61 | 64 |
No Takeover | 2 | 1 | 27 | 24 |
Total | 88 | 88 | 88 | 88 |
The effect of movement is significant on the MaxSWA during takeover (F(1, 34) = 7.28, p < .01, η2 = .07). Participants turned more the wheel when facing a static obstacle (M = 56.61 degrees, SE = 3.98 degrees) than a moving one (M = 41.58 degrees, SE = 3.98 degrees). No effect of danger and NDRT alone, and interaction effects were not significant (p > .05).
5.4 Physiological response
5.4.1 Skin Conductance Response: Peak Amplitude. The data analysis shows a significant effect of the movement of obstacles (F(1, 32) = 4.68, p < .05, η2 = .02) and the NDRT (F(1, 32) = 8.76, p = .01, η2 = .13) on the peak amplitude of SCRs. The peak amplitude was larger when reacting to static obstacles (M = 2.31 μ S, SE = 0.24 μ S) than to moving ones (M = 1.88 μ S, SE = 0.24 μ S). Also, the SCR peak amplitude was smaller for participants who engage in the NDRT (M = 1.46 μ S, SE = 0.30 μ S) than for those in the control group (M = 2.73 μ S, SE = 0.30 μ S). No effect of danger alone and interaction effects were found on the peak amplitude of SCRs (p > .05).
5.4.2 Skin Conductance Response: Rise Time. The data analysis showed a significant effect of the movement of obstacles on the rise time of SCRs (F(1, 32) = 4.50, p < .05, η2 = .03). The rise time of SCRs was shorter when facing moving obstacles (M = 1.74 s, SE = .14 s) than static ones (M = 2.10 s, SE = .14 s). No effect of danger, NDRT and interaction effects were found on the rise time of SCRs (p > .05).
5.4.3 Heart rate. The data analysis only shows a significant interaction effect of danger and NDRT on drivers’ heart rate during takeover periods (F(1, 73) = 6.83, p < .05, η2 = .01). This interaction effect is illustrated in Figure 5. When participants were engaged in the cognitive NDRT, the danger of obstacles did not influence drivers’ change in HR, while it had an influence on participants that did not engage in the task. For the latter, the change in HR was higher when facing dangerous obstacles than non-dangerous ones. No effect of danger, movement and NDRT alone was found, as well as no other significant interaction effect (p > .05).

6 DISCUSSION
6.1 Control of the experimental manipulation
One challenge of this study was to manipulate the takeover situation with regard to the danger and movement of obstacles triggering the TOR. Overall, the obstacles were considered realistic (around 5 out of 7 for each obstacle), with the frog considered the least realistic one.
On one hand, the manipulation of the takeover situation was successful according to participants’ subjective ratings on the danger of obstacles. The experimental design included four different takeover situations caused by obstacles of increasing levels of danger. The deer and the traffic cone were rated as more dangerous than the frog and the can, as desired in our experimental design. The moving obstacles (deer and frog) were rated as more dangerous than the static ones (cone and can). The unpredictable aspect of their movement might explain this higher danger perception felt by the participants.
On the other hand, the manipulation of the driver's cognitive state was successful. Participants in the manipulation group engaged correctly in the verbal cognitive NDRT, counting an average of one number every three seconds. They reported having a higher workload during the experiment regarding scores of the NASA-TLX questionnaire, which was confirmed by a higher heart rate compared to the control group. We can consider that the participants who engaged in the verbal cognitive NDRT had a higher workload throughout the drive and thus before the takeover situations. With the exception of the cognitive state, all participants were in the same physical condition when a takeover was required, as they were not performing any motor activity and were all expected to look at the vehicle environment.
6.2 Situation awareness
Data analysis showed a main effect of the obstacle's movement on both measures of SA. When facing a moving obstacle (crossing from the side of the road), participants reported having a lower SA. As hypothesised, the fact that the obstacles could move when the TOR was triggered may have made the situation more unstable and variable [17, 20, 48]. However, drivers took less time to report having understood the situation when faced with moving obstacles. The fact that obstacles could move probably led them to take less time in the steps that constitute SA (perception, comprehension, projection) before decision-making, so that they would be ready more quickly to take over control if needed, depending on the obstacle's movement.
The danger of obstacles alone only had an effect on the time participants took to understand the situation and press the button. Since they had to decide whether to regain control of the vehicle based on the potential of the obstacles’ danger, it is coherent to find significant differences in this measure. Participants were quicker to report having understood the situation after the TOR when faced with non-dangerous obstacles. It might have been easier and more straightforward to evaluate the potential of danger of non-dangerous obstacles, and thus led them to spend less time in SA stages (perception, comprehension, projection). However, the exposure to dangerous obstacles showed no influence on the driver's subjective SA. It may only have an impact on the time needed to understand a situation, and not necessarily on understanding quality.
Interestingly, engaging in a verbal cognitive NDRT did not have any effect on SA in this study. Results are different from previous literature which usually show a negative effect of NDRT engagement on SA [4, 56]. This nil effect of the NDRT engagement might be explained by the fact that all drivers had their gaze on the driving environment, regardless of their experimental condition. They might have collected the same amount of information, whether engaged in the NDRT or not.
6.3 Takeover behaviour of drivers
The data analysis showed a significant effect of the movement of obstacles on takeover performance measures of RT. Interestingly, the drivers took less time to report having understood the situation (TUS, see above) but more time to actually take over control when the obstacle was moving. They might have been quicker in the early stages of SA (perception and comprehension), but longer in the later stages (projection) and in the decision-making before doing the appropriate action. In other words, they might have taken more time to choose the best option to avoid the moving obstacles (steering or braking). Again, the unpredictable aspect of the obstacle's movement might have delayed the actual takeover. Also, drivers turned more the steering wheel in takeover situations involving static obstacles. This suggests that the majority of participants have chosen to avoid static obstacles, especially the traffic cone that could cause damage to the vehicle. Results suggest that RT and MaxSWA should be used to assess the driver behavior (and takeover performance) in takeover situations involving different obstacle types.
Besides, a significant effect of danger was found on RT. Drivers took over faster the control of the vehicle when facing dangerous obstacles. It is the opposite pattern as the one observed for the movement of the obstacle: drivers took more time to report having understood the situation but actually took over faster control when faced with dangerous obstacles. They probably took longer in the first stages of the SA because the obstacles were dangerous and it was more difficult to decide. Having taken more time to decide, the dangerous nature of the obstacles caused them to take control more quickly in order to avoid the danger. This shows that the dangerousness of a situation can cause an individual to reduce his/her decision time. However, it is difficult to judge whether the takeover quality was lower when a dangerous obstacle was in front of them, as no effect was found on the MaxSWA.
Engaging in the verbal cognitive NDRT also showed a significant effect on response time. Participants who had to engage in the NDRT took on average half a second more to take over control than participants who did not engage in the NDRT. The effect of NDRT on takeover performance is in line with previous studies [15, 34, 39, 51, 57, 59]. In particular, most of the previous studies showed an impairment of takeover performance when the NDRT is both visually and manually demanding and asks the driver to turn away the gaze from the road [15, 24, 39, 51, 57]. [51] found that the takeover performance when engaging in an auditory-vocal task was comparable to a baseline without any task. However, the significant result obtained in our study suggests that it might not be the case, in addition to results reported by [34] who also found a negative effect of a verbal cognitive task (twenty question task) on the mean speed after the takeover. Hence, further studies should be conducted to confirm the effect of engaging in a verbal cognitive NDRT on takeover performance.
6.4 Physiological state of drivers during takeovers
As for measures of SA and performance, an effect of the movement of obstacles was found on measures of skin conductance responses. After facing moving obstacles, the drivers’ rise time was shorter but the peak amplitude was smaller. This found pattern was not expected since a short rise time is usually associated with a high peak amplitude [3, 13], especially after the presentation of a stressful stimulus. Thus, facing moving obstacles is probably not related to a single increase in stress. The pattern found needs to be confirmed in further studies and could be an interesting measure to distinguish different stages of SA. Also, a recent study has shown that this can be a marker of hazard anticipation depending on driving experience [8], which goes in the same direction than in our study. Besides, no effect was found for the danger of obstacles on SCR measures. We would have expected a higher and quicker increase of the skin conductance when facing dangerous stimuli, as it quantifies the physiological response to a stressful event [9] or to a situation inducing fear of physical danger [12]. One possible explanation for this nil effect is that the feeling of danger was not strong enough. Indeed, the participants knew that they were in a simulation and that the obstacles could not harm them and cause damages to the vehicle. Besides, no effect of both movement and danger of obstacles was found on the average heart rate. Even if 10 seconds are enough for a reliable measurement of the heart rate [45], it might not be the most appropriate measure to quantify an individual's physiological response to a stressful event in such a short period.
For the effect of the NDRT on the physiological response of drivers, data analysis showed that participants who engaged in the NDRT showed a smaller increase in the skin conductance response during the takeover situations, compared to participants who did not engage in the task. This result differs from previous studies which showed that higher mental workload was associated with higher SCR amplitude [29], whereas [46] did not find any effect of increased workload on that measure. Hence, the effect of workload on skin conductance responses would need further investigation.
6.5 Summary of results and implications for human-vehicle interaction
To summarize, we can say that:
- (H1) is validated for SA and takeover performance measures, but not for the physiological measures. Indeed, a negative effect of the obstacles’ movement was found on SA and takeover performance. When obstacles are moving, drivers have a lower SA and take less time (not enough?) to understand the situation, and take more time (too much?) to take over control of the vehicle. It suggests that drivers spend more time in higher stages of SA (projection) when the obstacle was moving. Also, the steering wheel angle was greater for moving obstacles, but this reflects the need to avoid the obstacle rather than poor takeover control. For the physiological response of the driver, moving obstacles generated a shorter rise time but a small peak amplitude of the SCRs. This unexpected found pattern should be confirmed in further studies.
- (H2) is refuted since an exposure to dangerous obstacles showed an effect on the time to understand the situation and the time to take over control (response time). When obstacles are considered dangerous, drivers take more time (too much?) to understand the situation (lower SA stages), and less time (not enough?) to take over control of the vehicle, thus reducing the time allocated to decision-making process (higher SA stages). On the other hand, no effect of the danger was found on the comprehension of the situation (subjective SA), the steering wheel angle or the physiological responses. The results still do suggest that more research on the danger of obstacles might be interesting, as this factor varies greatly in a real-world driving environment (e.g., pedestrian vs. roadside trash).
- (H3): the hypothesis on the engagement in an NDRT is confirmed for response time to take over control (longer when engaged in a cognitive verbal task). However, the hypothesis is invalidated for the other measures, as no effect of the driver's mental state was found on the physiological state during takeover situations or on SA.
Overall, these results suggest that the type of obstacles/entities present in the vehicle's environment, and particularly their movement, may have an effect on situation awareness, but also on the time required to understand the situation and then take over control when necessary. In order to maintain drivers’ awareness and optimise the takeover quality, it would be helpful to provide the driver with some information about the nature of obstacles and other road users around the car, via human-vehicle interfaces (HMIs) [5, 6, 35]. If one wants to indicate potential movement and danger (or their severity) directly to the driver via HMIs in the vehicle, we thought of two possibilities. The first would be to do it directly on the screen of the vehicle's central console where a map of the environment would be displayed with the entities present around the vehicle. The danger (i.e. the level of severity) of each obstacle could be indicated by visual indicators such as colours (green/orange/red) or numbers above the obstacle (e.g. from 1 to 5). The movement of the obstacle could be indicated by an arrow pointing in the direction of the likely movement, or by a cross if the detected obstacle is static. These indications could also be done in the vehicle through augmented reality techniques, by displaying them on the windscreen or side windows.
In parallel to this, the results suggest that it would also be useful for an intelligent driver monitoring system to detect the type of NDRT the driver is engaged in as this has an impact on response time. Even if the driver's eyes are directed towards the windshield, his or her mental state is important to take into account, which can be predicted with physiological indicators used as inputs of machine learning models [36, 37, 47]. Depending on the drivers’ direction of gaze (handheld device or windscreen) and their cognitive state (high or low workload), the intelligent system could adapt the location, type and amount of information transmitted to the driver, allowing for greater adaptability and comfort for the driver during conditionally automated driving phases [14].
6.6 Limitations and further work
In the experimental design, the movement variable was confounded by the fact that the moving objects were living and the non-moving ones were non-living. It is possible that the participants’ responses might relate to the movement, the living nature, or both. The living nature could be included in the experimental design of a future study. Also, the color and the size of obstacles might have affected the ability to see the obstacle when TOR is triggered. The effects found in this study could have been different if other obstacles would have been selected in the design. For this, it would be interesting to test the same experimental design but with other obstacles of the same type: bear or dog instead of deer, and rock or wood on the road instead of a traffic cone.
With regard to the driver's mental state, it would be more appropriate to further distinguish between the different types of (cognitively demanding) tasks in a single study, as different results have been found in the literature. Although the induction of mental load was validated by this task, it could also be perceived as long and boring by some participants. Other tasks performed over a shorter period of time may be more appropriate and realistic for further research.
7 CONCLUSION
In this study, 88 participants monitored the environment of a conditionally automated vehicle for 20 minutes. They responded to four takeover requests triggered by different obstacles, which varied in movement and danger: a deer, a cone, a frog, and a can. Half of the participants were asked to engage in a verbal cognitive non-driving related task (NDRT) throughout the driving session (count backwards in steps of two). The results of this study indicate that moving obstacles are perceived as more dangerous by drivers. The movement of the obstacles also has an influence on the situation awareness and response time during the takeover process. When faced with moving obstacles, drivers reported lower situational awareness, took less time to understand the situation, but took more time to take over control. The danger of the obstacle (i.e., the potential to cause damage) had a significant effect on the time drivers took to understand the situation, but also on the response time for the actual takeover. When faced with dangerous obstacles, drivers took more time to report having understood the situation but less time to do the actual takeover, suggesting a reduction of the decision-making process duration. The engagement of drivers in a verbal cognitive NDRT only showed a significant effect on takeover response time. The results of this study suggest that both the driving situation (i.e., movement and danger of entities in the vehicle surroundings) and the driver's cognitive state should be known at any time, especially before a takeover request occurs. Based on this, the location, the quantity, and the type of information conveyed to the driver through in-car human-vehicle interfaces could be smartly adapted. This would contribute to maintaining drivers’ situation awareness and takeover performance in conditionally automated driving.
ACKNOWLEDGMENTS
This work was supported and financed by the Hasler Foundation in the framework of the AdVitam project. The authors would like to thank in particular Océane Minot, Laura Rege-Colet, Stanislav Riss and Christian Schoepfer, for their great help in designing the experiment and collecting the data.
REFERENCES
- Shubham Agrawal and Srinivas Peeta. 2021. Evaluating the impacts of situational awareness and mental stress on takeover performance under conditional automation. Transportation research part F: traffic psychology and behaviour 83 (2021), 210–225.
- Pavlo Bazilinskyy and Joost de Winter. 2018. Crowdsourced Measurement of Reaction Times to Audiovisual Stimuli With Various Degrees of Asynchrony. Human factors 60, 8 (Dec 2018), 1192–1206. https://doi.org/10.1177/0018720818787126
- Wolfram Boucsein. 2012. Electrodermal Activity (2 ed.). Springer US.
- Mercedes Bueno, Ebru Dogan, Fouad Hadj-Selem, Éric Monacelli, Serge Boverie, and Anne Guillaume. 2016. How different mental workload levels affect the take-over control after automated driving. 2016 IEEE 19th International Conference on Intelligent Transportation Systems (ITSC) (2016), 2040–2045.
- Marine Capallera, Peïo Barbé-Labarthe, Leonardo Angelini, Omar Abou Khaled, and Elena Mugellini. 2019. Convey situation awareness in conditionally automated driving with a haptic seat. Proceedings of the 11th International Conference on Automotive User Interfaces and Interactive Vehicular Applications: Adjunct Proceedings (2019).
- Marine Capallera, Emmanuel de Salis, Quentin Meteier, Leonardo Angelini, Stefano Carrino, Omar Abou Khaled, and Elena Mugellini. 2019. Secondary task and situation awareness, a mobile application for conditionally automated vehicles. Proceedings of the 11th International Conference on Automotive User Interfaces and Interactive Vehicular Applications: Adjunct Proceedings (2019).
- Marine Capallera, Quentin Meteier, Emmanuel de Salis, Leonardo Angelini, Stefano Carrino, Omar Abou Khaled, and Elena Mugellini. 2019. Owner Manuals Review and Taxonomy of ADAS Limitations in Partially Automated Vehicles. In Proceedings of the 11th International Conference on Automotive User Interfaces and Interactive Vehicular Applications (Utrecht, Netherlands) (AutomotiveUI ’19). Association for Computing Machinery, New York, NY, USA, 156–164. https://doi.org/10.1145/3342197.3344530
- Theresa Chirles, Johnathon P. Ehsani, Neale Kinnear, and Karen E Seymour. 2021. Skin Conductance Responses of Learner and Licensed Drivers During a Hazard Perception Task. Frontiers in Psychology 12 (2021).
- H. Cohen, M. Kotler, M. Matar, Z. Kaplan, and Y. Cassuto. 1998. Analysis of heart rate variability in posttraumatic stress disorder patients in response to a trauma-related reminder. Biological Psychiatry 44 (1998), 1054–1059.
- C. Collet, A. Clarion, M. Morel, A. Chapon, and C. Petit. 2009. Physiological and behavioural changes associated to the management of secondary tasks while driving. Applied Ergonomics 40, 6 (Nov. 2009), 1041–1046. https://doi.org/10.1016/j.apergo.2009.01.007
- Benjamin Cortens, Blair Nonnecke, and Lana M. Trick. 2019. Effect of Alert Presentation Mode and Hazard Direction on Driver Takeover from an Autonomous Vehicle. Proceedings of the 10th International Driving Symposium on Human Factors in Driver Assessment, Training and Vehicle Design: driving assessment 2019 (2019).
- B. Cuthbert, P. Lang, C. Strauss, D. Drobes, C. Patrick, and M. Bradley. 2003. The psychophysiology of anxiety disorder: fear memory imagery.Psychophysiology 40 3 (2003), 407–22.
- Michael E. Dawson, Anne M. Schell, and Dianne L Filion. 2007. The Electrodermal System. In Handbook of psychophysiology, J.T. Cacioppo, L.G. Tassinary, and G. Berntson (Eds.). Cambridge University Press, Chapter 7, 159–181. https://doi.org/10.1017/CBO9780511546396.007
- Emmanuel de Salis, Marine Capallera, Quentin Meteier, Leonardo Angelini, Omar Abou Khaled, Elena Mugellini, Marino Widmer, and Stefano Carrino. 2020. Designing an AI-Companion to Support the Driver in Highly Autonomous Cars. In HCI.
- Na Du, Jinyong Kim, Feng Zhou, Elizabeth Pulver, Dawn M. Tilbury, Lionel Peter Robert, Anuj K. Pradhan, and X. Jessie Yang. 2020. Evaluating Effects of Cognitive Load, Takeover Request Lead Time, and Traffic Density on Drivers’ Takeover Performance in Conditionally Automated Driving. 12th International Conference on Automotive User Interfaces and Interactive Vehicular Applications (2020).
- M. Endsley. 1988. Situation awareness global assessment technique (SAGAT). Proceedings of the IEEE 1988 National Aerospace and Electronics Conference (1988), 789–795 vol.3.
- Mica R. Endsley. 1995. Toward a Theory of Situation Awareness in Dynamic Systems. Human Factors: The Journal of the Human Factors and Ergonomics Society 37, 1 (Mar 1995), 32–64. https://doi.org/10.1518/001872095779049543
- Alexandra Fernandes and Per Øivind Braarud. 2015. Exploring Measures of Workload, Situation Awareness, and Task Performance in the Main Control Room. Procedia Manufacturing 3 (2015), 1281–1288. https://doi.org/10.1016/j.promfg.2015.07.273 6th International Conference on Applied Human Factors and Ergonomics (AHFE 2015) and the Affiliated Conferences, AHFE 2015.
- Kelly Funkhouser and Frank Drews. 2016. Reaction Times When Switching From Autonomous to Manual Driving Control: A Pilot Investigation. Proceedings of the Human Factors and Ergonomics Society Annual Meeting 60, 1 (2016), 1854–1858. https://doi.org/10.1177/1541931213601423 arXiv:https://doi.org/10.1177/1541931213601423
- V. Greco and D. Roger. 2003. Uncertainty, stress, and health. Personality and Individual Differences 34 (2003), 1057–1068.
- S. G. Hart. 2006. Nasa-Task Load Index (NASA-TLX); 20 Years Later. Proceedings of the Human Factors and Ergonomics Society Annual Meeting 50 (2006), 904 – 908.
- Sandra G. Hart and Lowell E. Staveland. 1988. Development of NASA-TLX (Task Load Index): Results of Empirical and Theoretical Research. In Advances in Psychology, Peter A. Hancock and Najmedin Meshkati (Eds.). Human Mental Workload, Vol. 52. North-Holland, 139–183. https://doi.org/10.1016/S0166-4115(08)62386-9
- SAE International. 2018. Taxonomy and Definitions for Terms Related to Driving Automation Systems for On-Road Motor Vehicles.
- Oliver Jarosch, Hanna Bellem, and Klaus Bengler. 2019. Effects of Task-Induced Fatigue in Prolonged Conditional Automated Driving. Human Factors: The Journal of Human Factors and Ergonomics Society 61 (2019), 1186 – 1199.
- David B. Kaber, Yu Zhang, Sangeun Jin, Prithima Mosaly, and Megan Garner. 2012. Effects of hazard exposure and roadway complexity on young and older driver situation awareness and performance. Transportation Research Part F-traffic Psychology and Behaviour 15 (2012), 600–611.
- Roger Kirk. 2013. Latin square and related designs. In Experimental Design: Procedures for the Behavioral Sciences (4 ed.). SAGE Publications, Inc., Thousand Oaks, California. https://doi.org/10.4135/9781483384733
- David R. Large, Gary Burnett, Davide Salanitri, Anneka Lawson, and Elizabeth Box. 2019. A Longitudinal Simulator Study to Explore Drivers’ Behaviour in Level 3 Automated Vehicles. In Proceedings of the 11th International Conference on Automotive User Interfaces and Interactive Vehicular Applications. ACM, 222–232. https://doi.org/10.1145/3342197.3344519
- Nengchao Lyu, Lian Xie, Chaozhong Wu, Qiang Fu, and Chao Deng. 2017. Driver's Cognitive Workload and Driving Performance under Traffic Sign Information Exposure in Complex Environments: A Case Study of the Highways in China. International Journal of Environmental Research and Public Health 14 (2017).
- Megan K. MacPherson, D. Abur, and C. Stepp. 2017. Acoustic Measures of Voice and Physiologic Measures of Autonomic Arousal during Speech as a Function of Cognitive Load.Journal of voice : official journal of the Voice Foundation 31 4 (2017), 504.e1–504.e9.
- Dominique Makowski, Tam Pham, Zen J. Lau, Jan C. Brammer, François Lespinasse, Hung Pham, Christopher Schölzel, and S. H. Annabel Chen. 2021. NeuroKit2: A Python toolbox for neurophysiological signal processing. Behavior Research Methods (02 Feb 2021). https://doi.org/10.3758/s13428-020-01516-y
- Bruce Mehler, B. Reimer, and J. Coughlin. 2012. Sensitivity of Physiological Measures for Detecting Systematic Variations in Cognitive Demand From a Working Memory Task. Human Factors: The Journal of Human Factors and Ergonomics Society 54 (2012), 396 – 412.
- Bruce Mehler, Bryan Reimer, Joseph Coughlin, and Jeffery Dusek. 2009. The Impact of Incremental Increases in Cognitive Workload on Physiological Arousal and Performance in Young Adult Drivers. Transportation Research Record: Journal of the Transportation Research Board 2138 (Dec. 2009), 6–12. https://doi.org/10.3141/2138-02
- Bruce Mehler, B. Reimer, and Y. Wang. 2017. A Comparison of Heart Rate and Heart Rate Variability Indices in Distinguishing Single-Task Driving and Driving Under Secondary Cognitive Workload.
- Natasha Merat, A. Hamish Jamson, Frank C. H. Lai, and Oliver M. J. Carsten. 2012. Highly Automated Driving, Secondary Task Performance, and Driver State. Human Factors: The Journal of Human Factors and Ergonomics Society 54 (2012), 762 – 771.
- Quentin Meteier, Marine Capallera, Emmanuel de Salis, Andreas Sonderegger, Leonardo Angelini, Stefano Carrino, Omar Abou Khaled, and Elena Mugellini. 2020. The Effect of Instructions and Context-Related Information about Limitations of Conditionally Automated Vehicles on Situation Awareness. 12th International Conference on Automotive User Interfaces and Interactive Vehicular Applications (2020).
- Quentin Meteier, Marine Capallera, Simon Ruffieux, Leonardo Angelini, Omar Abou Khaled, Elena Mugellini, Marino Widmer, and Andreas Sonderegger. 2021. Classification of Drivers’ Workload Using Physiological Signals in Conditional Automation. Frontiers in Psychology 12 (2021).
- Quentin Meteier, Emmanuel de Salis, Marine Capallera, Marino Widmer, Leonardo Angelini, Omar Abou Khaled, Andreas Sonderegger, and Elena Mugellini. 2021. Relevant Physiological Indicators for Assessing Workload in Conditionally Automated Driving, Through Three-Class Classification and Regression. In Frontiers in Computer Science.
- Brian Mok, Mishel Johns, David Miller, and Wendy Ju. 2017. Tunneled in: Drivers with active secondary tasks need more time to transition from automation. In Proceedings of the 2017 CHI Conference on Human Factors in Computing Systems. 2840–2844.
- Frederik Naujoks, Christian Purucker, Katharina Wiedemann, and Claus Marberger. 2019. Noncritical State Transitions During Conditionally Automated Driving on German Freeways: Effects of Non–Driving Related Tasks on Takeover Time and Takeover Quality. Human Factors: The Journal of Human Factors and Ergonomics Society 61 (2019), 596 – 613.
- Thanh Nguyen, Chee Peng Lim, Ngoc Duy Nguyen, Lee Gordon-Brown, and Saeid Nahavandi. 2019. A Review of Situation Awareness Assessment Approaches in Aviation Environments. (2019), 16.
- Julie Paxion, Edith Galy, and Catherine Berthelon. 2014. Mental workload and driving. Frontiers in Psychology 5 (2014).
- Bryan Reimer and Bruce Mehler. 2013. The Effects of a Production Level “Voice-Command” Interface on Driver Behavior: Summary Findings on Reported Workload, Physiology, Visual Attention, and Driving Performance. (2013), 20.
- Janette Rose, Chris Bearman, Anjum Naweed, and Jillian Dorrian. 2019. Proceed with caution: using verbal protocol analysis to measure situation awareness. Ergonomics 62, 1 (2019), 115–127. https://doi.org/10.1080/00140139.2018.1527951 arXiv:https://doi.org/10.1080/00140139.2018.1527951PMID: 30265217.
- Marlene Susanne Lisa Scharfe, Kathrin Zeeb, and Nele Rußwinkel. 2020. The Impact of Situational Complexity and Familiarity on Takeover Quality in Uncritical Highly Automated Driving Scenarios. Inf. 11 (2020), 115.
- F Shaffer, S Shearman, Z Meehan, N Gravett, and H Urban. 2019. The promise of ultra-short-term (UST) heart rate variability measurements: a comparison of Pearson product-moment correlation coefficient and limits of agreement (LoA) concurrent validity criteria. Physiological Recording Technology and Applications in Biofeedback and Neurofeedback, eds D. Moss and F. Shaffer (Oakbrook Terrace, IL: Association for Applied Psychophysiology and Biofeedback) (2019), 214–220.
- Yoshihiro Shimomura, Takumi Yoda, Koji Sugiura, Akinori Horiguchi, Koichi Iwanaga, and Tetsuo Katsuura. 2008. Use of frequency domain analysis of skin conductance for evaluation of mental workload.Journal of physiological anthropology 27 4 (2008), 173–7.
- Erin Treacy Solovey, Marin Zec, Enrique Abdon Garcia Perez, B. Reimer, and Bruce Mehler. 2014. Classifying driver workload using physiological and driving performance data: two field studies. Proceedings of the SIGCHI Conference on Human Factors in Computing Systems (2014).
- Richard M Taylor. 2017. Situational awareness rating technique (SART): The development of a tool for aircrew systems design. In Situational awareness. Routledge, 111–128.
- John R Treat, Nicholas S Tumbas, Stephen T McDonald, David Shinar, Rex D Hume, RE Mayer, RL Stansifer, and N John Castellan. 1979. Tri-level study of the causes of traffic accidents: final report. Executive summary.Technical Report. Indiana University, Bloomington, Institute for Research in Public Safety.
- Arie P. van den Beukel and Mascha C. van der Voort. 2017. How to assess driver's interaction with partially automated driving systems – A framework for early concept assessment. Applied Ergonomics 59 (Mar 2017), 302–312. https://doi.org/10.1016/j.apergo.2016.09.005
- Bernhard Wandtner, Nadja Schömig, and Gerald J. Schmidt. 2018. Effects of Non-Driving Related Task Modalities on Takeover Performance in Highly Automated Driving. Human Factors: The Journal of Human Factors and Ergonomics Society 60 (2018), 870 – 881.
- Bradley W. Weaver and Patricia R. Delucia. 2020. A Systematic Review and Meta-Analysis of Takeover Performance During Conditionally Automated Driving.Human factors (2020), 18720820976476.
- Christopher D. Wickens. 2008. Multiple Resources and Mental Workload. Human Factors 50, 3 (2008), 449–455. https://doi.org/10.1518/001872008X288394 arXiv:https://doi.org/10.1518/001872008X288394PMID: 18689052.
- Allan F. Williams and Veronika I. Shabanova. 2003. Responsibility of drivers, by age and gender, for motor-vehicle crash deaths. Journal of Safety Research 34, 5 (2003), 527–531. https://doi.org/10.1016/j.jsr.2003.03.001
- E. Won and Y. Kim. 2016. Stress, the Autonomic Nervous System, and the Immune-kynurenine Pathway in the Etiology of Depression. Current Neuropharmacology 14 (2016), 665 – 673.
- Felix Wulf, Kathrin Zeeb, Maria Rimini-Doring, Marc Arnon, and Frank Gauterin. 2013. Effects of human-machine interaction mechanisms on situation awareness in partly automated driving. IEEE Conference on Intelligent Transportation Systems, Proceedings, ITSC, 2012–2019. https://doi.org/10.1109/ITSC.2013.6728525
- Sol Hee Yoon and Yong Gu Ji. 2019. Non-driving-related tasks, workload, and takeover performance in highly automated driving contexts. Transportation Research Part F: Traffic Psychology and Behaviour 60 (2019), 620–631. https://doi.org/10.1016/j.trf.2018.11.015
- Mark S. Young, Karel A. Brookhuis, Christopher D. Wickens, and Peter A. Hancock. 2015. State of science: mental workload in ergonomics. Ergonomics 58, 1 (Jan. 2015), 1–17. https://doi.org/10.1080/00140139.2014.956151
- Kathrin Zeeb, A. Buchner, and M. Schrauf. 2016. Is take-over time all that matters? The impact of visual-cognitive load on driver take-over quality after conditionally automated driving.Accident; analysis and prevention 92 (2016), 230–9.
This work is licensed under a Creative Commons Attribution International 4.0 License.
IHM '23, April 03–06, 2023, TROYES, France
© 2023 Copyright held by the owner/author(s).
ACM ISBN 978-1-4503-9824-4/23/04.
DOI: https://doi.org/10.1145/3583961.3583966