Abstract
We investigated the influence of visual sensitivity on the performance of an imitation task with the robot R1 in its virtual and physical forms. Virtual and physical embodiments offer different sensory experience to the users. As all individuals respond differently to their sensory environment, their sensory sensitivity may play a role in the interaction with a robot. Investigating how sensory sensitivity can influence the interactions appears to be a helpful tool to evaluate and design such interactions. Here we asked 16 participants to perform an imitation task, with a virtual and a physical robot under conditions of full and occluded visibility, and to report the strategy they used to perform this task. We asked them to complete the Sensory Perception Quotient questionnaire. Sensory sensitivity in vision predicted the participants’ performance in imitating the robot’s upper limb movements. From the self-report questionnaire, we observed that the participants relied more on visual sensory cues to perform the task with the physical robot than on the virtual robot. From these results, we propose that a physical embodiment enables the user to invest a lower cognitive effort when performing an imitation task over a virtual embodiment. The results presented here are encouraging that following this line of research is suitable to improve and evaluate the effects of the physical and virtual embodiment of robots for applications in healthy and clinical settings.
1 Introduction
Nowadays, the use of social, interactive robots is increasing in our daily life. How to improve the interaction between one or more users and one or several robots is one of the main questions of the human–robot interaction research field. Interindividual differences in the acceptance of the robot as an interaction partner have often been reported [1,2,3,4,5]. One critical dimension along which the users differ is their sensory sensitivity. For example, some individuals would suffer from exposure to background noise in an open-space environment or would be affected by bright lights in a shopping mall, whereas others would be less disturbed. Sensory sensitivity is mainly discussed and defined in individuals diagnosed with autism spectrum disorder (ASD) as a hypo- or hyper-reactivity to sensory stimuli [6]. However, the sensitivity to sensory stimuli can be found in typical population as well [7], although this too was found to be correlated with sub-diagnostic autistic traits [8]. In ref. [8], the authors discussed that, for auditory inputs, in the range of audible frequencies, individuals’ “comfort zones” differ and would be narrower for individuals diagnosed with ASD. We believe that sensory sensitivity is critical in human–robot interaction, as sensory information plays a major role in interactions with the environment, including social interactions [9]. The use of vision enables people to observe the interaction partner’s expressions and bodily behavior. Hearing is used to analyze emotion in the speaker’s voice or to follow instructions. In addition, robots are a complex source of sensory information, as they vary in embodiment types (e.g., humanoid or not, physical or virtual), display mechanical parts or LEDs, and produce noise from their motors, mechanical joints, and loudspeakers.
To our knowledge, only a few studies investigated the role of sensory sensitivity in the acceptance of social robots. In clinical settings, sensory sensitivity in vision and proprioception was investigated as a predictor of performance in an interaction with a robot (in imitation [3], emotion recognition [10], and joint attention [11]). In ref. [11], 11 children diagnosed with ASD were asked to perform a joint attention task with the robot Nao (Softbank Robotics). The results showed a relationship between their reaction times and their proprioception and visual sensitivity. However, these results were obtained during a single interaction with the robot, with few joint attention trials. Similarly, in ref. [10], 19 children and adults diagnosed with ASD were asked to identify four emotions on different artificial agents (two robots, a virtual agent, and a human). A relationship between correct classification of facial expression of emotion and proprioception and visual sensitivity was found. Finally, in ref. [3], 12 children diagnosed with ASD did an imitation training with the robot Nao. Sensory sensitivity in vision and proprioception was found to predict improvement in imitation during the sessions. Overall, these studies show that in individuals diagnosed with ASD, visual and proprioception sensitivity affected the success of the interaction (e.g., training imitation) and in emotion recognition [10]. However, little is known about the impact of visual sensitivity in general (healthy) population on engagement with a robot. This information is crucial in order to make comparisons with clinical populations. In typically developed adults, the auditory sensitivity was shown to have an impact on the performance in a Stroop task with the Tiago robot [4]. In this single case experiment, the authors manipulated visual and auditory stimuli from the robot (e.g. movements and speech volume) to make it appear neutral, encouraging, or stressing. The participants were screened for their sensory sensitivity in audition and vision. Individuals who generally seek auditory sensation or who do not detect changes in auditory information performed better with a stressing and an encouraging robot, compared with the neutral robot. The results of this work demonstrate an impact of the sensory sensitivity of a typical individual in a cognitive task done with a robot, and that manipulating the robot’s behavior in auditory and visual cues can help improve participants’ performance.
In this context, it is important to further understand the impact of users’ sensory sensitivity on their interactions with social robots. These considerations will be impactful for both healthy and clinical populations. Indeed, the more attuned and engaging the robot is, the more benefits the interaction might bring [12,13,14], and sensory sensitivity might have an impact on engagement. In ref. [12,14], engagement with the robot was found to be beneficial during stroke rehabilitation therapy. The engagement with the robot helped the users perform exercises for longer periods. In long-term interactions with a robot, the engagement with the user is often discussed as a limitation and a point to improve in future work, as observed in cases diagnosed with ASD (see ref. [15]). Therapists, during interviews reported in ref. [13], highlighted the need for engagement during therapies including a social robot. Therefore, maximizing the engagement and interest of the users in the interaction can improve their performance and avoid premature withdrawal from the training program. We believe that studying the sensory sensitivity of users of social robots, especially in socially assistive robotics, could improve engagement and commitment to interaction.
In the framework of a collaboration between the Italian Institute of Technology and the Don Gnocchi Foundation, we tested the use of the R1 robot [16] (see Figure 1), as a training coach for upper limb rehabilitation in an imitation task with neurotypical young adults, in view of using the robot with elderly individuals in later phases of the project. We believe that this is a fundamental preliminary step, which provides a baseline comparison for tests with nonhealthy individuals. In a previous article [17], we investigated the differences in the participants’ engagement toward the robot and performance in the task when the robot was presented in its physical and virtual forms. Virtual agents are often used in stroke rehabilitation therapy, and we investigated which type of embodiment was the most engaging for participants. As expected from literature (see ref. [18,19] for reviews on the impact of the robot embodiment types), we observed that the physical form of R1 improved the engagement of our participants. We believe that real and virtual robots offer a different sensory experience to the user. For examples for visual cues, the flickering of the screen in which the virtual robot is displayed may interfere with the task for some sensitive participants, or similarly with bright lights from the LED display of the robots. In this article, we aimed to observe the influence of sensory sensitivity in vision on our participants’ performance in the imitation task, with regard to the different embodiments. With this study we aim to advance the knowledge of how sensory sensitivity can affect the interactions between a human and a robot partner. Investigating the difference between physical and virtual embodiments in regard to sensory sensibility was not done previously to our knowledge.
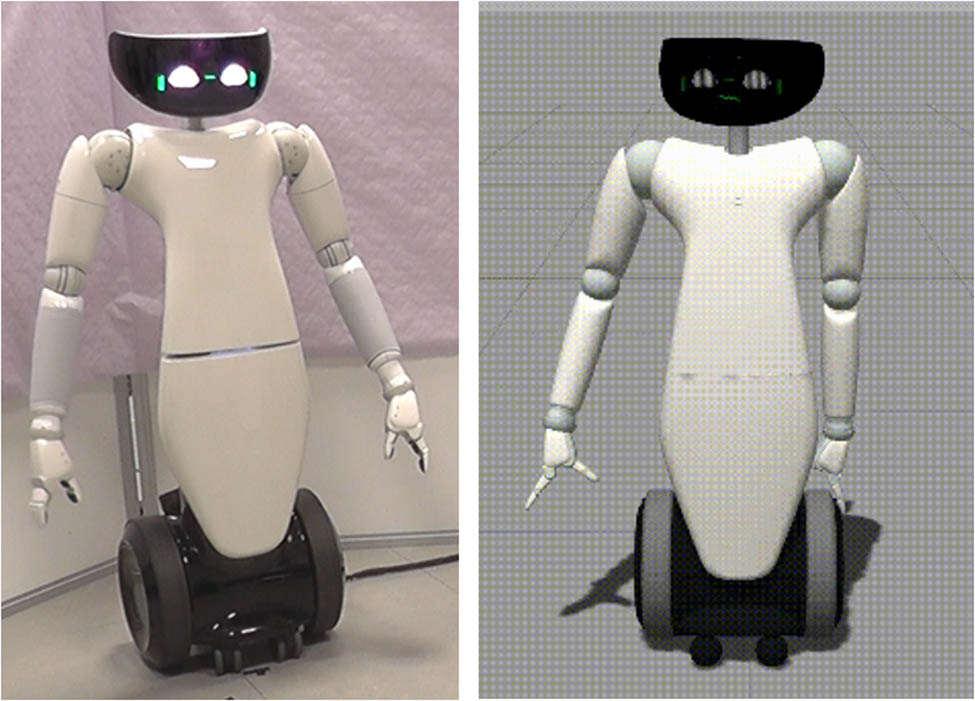
The robot R1 in its physical (left) and virtual (right) embodiments.
2 Methods
2.1 Robot and framework
We used the robot R1 [16], a 1.2 m-high humanoid capable of extending the torso height up to 1.35 m, with two arms of 8 degrees of freedom (Figure 1 [left]). The robot’s head is equipped with an Intel Real Sense RGB-D camera for depth sensing. We developed a realistic simulation of the robot in the environment Gazebo (Figure 1 [right]). The robot and its simulated version run on YARP [20], an open-source middleware in which a large variety of modules running on different operating systems can be interconnected.
The devised framework[1] used in this article retrieves human skeletons from RGB-D camera to analyze actions and to provide verbal feedback to the user. The framework and its modules are shown in Figure 2. First, we detect 2D human key points on the RGB image provided by the camera, using OpenPose [21], an open-source library for real-time multiperson 2D pose estimation. Key points are then reconstructed in 3D fashion, using the depth provided by the camera, with the classical pinhole camera model (i.e., skeletonRetriever). The retrieved skeleton undergoes an action recognition layer, which classifies the skeleton data in a predefined temporal window, using a Recurrent Neural Network with Long Short-Term Memory cells (i.e., actionRecognizer). We trained the network in a supervised fashion off-line, with pairs of temporal sequences of fixed length, including 2D joints of the upper body, and the corresponding class labels (abduction and random). At run time, if the predicted class has the label of the exercise to perform (i.e., abduction), we perform a finer analysis, by comparing the observed skeleton with a prerecorded template, moving coherently with the robot (i.e., motionAnalyzer). Observed and template skeletons are aligned spatially, considering the roto-translational offset between the bodies and, temporally, applying the Dynamic Time Warping to the 3D joint positions. We then perform a statistical analysis of the error in position between each component of the candidate and template skeletons and look for positive and negative tails in the error distribution, which reflect to a higher and lower range of motion, respectively. We also evaluate the speed performing the Fourier transform of each component of the joints under analysis: a positive difference in frequency relates to a skeleton moving slower than the template and a negative difference relates to a faster movement than the skeleton. Finally, the participant is associated with a score ranging from “0” (if the movement is completely wrong) to “1” (if no error is detected). Real-time verbal feedback is synthetized according to the protocol summarized in Table 1. For this article, the template is a prerecorded skeleton generated by a person performing the exercise with the robot and is normalized at run time to deal with differences in the body structure. However, there might be small differences between the template and the robot movement (in terms of amplitude and timing). To avoid such variations in future studies, a new version of the template was developed to now compute at run time the 3D configuration of the robot limbs directly from the robot’s joints.

The devised framework.
Verbal feedback delivered by the robot during the interaction
Detected error | Score | Verbal feedback |
---|---|---|
Action not recognized | 0.0 | Please put more effort |
df > 0 (<0) | 0.5 | Move the left arm faster (slower) |
ε x > 0 (<0) | 0.5 | Move the left arm more on the left (on the right) |
ε y > 0 (<0) | 0.5 | Move the left arm further up (further down) |
ε z > 0 (<0) | 0.5 | Move the left arm backward (forward) |
— | 1.0 | You are moving very well |
2.2 Experimental design
2.2.1 Participants
We recruited 16 participants (age M = 23.0, SD = 2.81; 7 males) via the web platform “join the science” of the Italian Institute of Technology. We sampled 16 participants due to the limited time of the robot availability and the long duration of the experiment and installation of the participants (around 1½ h per participant). Our study adhered to the ethical standards laid down in the Declaration of Helsinki and was approved by the local Ethics Committee (Comitato Etico Regione Liguria). Participants were compensated with 20€ for their participation. Only right-handed participants were recruited for the experiment. First, the participants were asked to imitate a movement with their left arm and choosing right-handed participants ensures us that dominance differences would not be an issue. Second, for the study reported in ref. [17] we also recorded the neural activity, for which it is typical to only select right-handed individuals to avoid potential variations in brain structure and functioning related to left-handedness. Participants did not report any previous familiarity with robots.
2.2.2 Design considerations
The imitation task has been chosen as it is common during typical rehabilitation therapy. Robots have been used in upper limb rehabilitation for stroke patients (see ref. [22] for a systematic review), for children with physical impairment in pediatric rehabilitation structures [23], or for children diagnosed with ASD to train body movement imitation [3,24]. In the typically developed population, the use of robots has been discussed to promote sports activities to prevent insufficient physical activity [25]. In our task, participants performed an imitation task with a physically embodied R1 robot and also with its virtual version. Critically, we introduced two additional conditions for both the physical and the virtual robot: one in which the robot was visible to the participants and one in which it was occluded. We were interested in the accuracy of continuation of the imitation rhythm in the absence of visual feedback [17]. Patients undergoing such therapy often suffer from sensitivity impairments, for example, vision deficiency is common after stroke or in the elderly. It is then relevant to investigate the relationship between sensory sensitivity in the context of rehabilitation with artificial agents. However, before recruiting patients in rehabilitation therapy to participate to this study, we wanted to test our setup with young healthy individuals. Indeed, it enables to verify the feasibility of the setup without recruiting vulnerable population.
2.2.3 Procedure
In this study, the participants were asked to do an imitation task with the R1 robot, in its physical and virtual embodiments. After receiving their informed consent, we gave the participants earplugs to wear to attenuate background and actuator noise, and we fitted them with an EEG equipment (EEG data are not the focus of this article, see ref. [17]). The setup is visualized in Figure 3. Two types of embodiment were presented in counterbalanced order to the participants, so that half of the participants interacted first with the physical robot and then with the virtual robot, and the other half interacted first with the virtual robot and then with the physical robot. The physical robot and the screen displaying the virtual robot were placed at the same distance from the participant. Before the experiment, the experimenters informed the participants of the procedure (the text provided to the participant can be found in the Supplementary Material). Participants had to perform abduction movements with the left arm following the robot’s instructions, during three conditions. First, they had to observe the robot’s arm performing the abduction movement (observation, see Figure 3 first row). This step acted as a training phase, as when a therapist demonstrates the movement to patients. Then they had to do the movement with the robot (visible imitation, see Figure 3 second row). Finally they had to do the movement with the robot with its arm occluded from the participant’s view (occluded imitation, see Figure 3 third row). The occlusion phase was added to evaluate how patients would perform in situations in which perceptual information processing is not complete. Instructions for these three conditions were provided by the experimenters before the experiment commenced. When the participant was interacting with the physical robot, one of the experimenters placed a panel in front of the robot arm at the onset of the occluded imitation condition. With the virtual robot, a virtual panel appeared in front of the robot’s arm. For each condition, the participants performed the movement with the robot eight times, and the sequence (i.e., observation – visible imitation – occluded imitation) was repeated six times for each embodiment type of the robot. During the visible imitation phase, the verbal feedback (see Table 1) was delivered approximately every three and half movements (around 18 s). Participants were allowed breaks between each sequence. When the six sequences were performed with the physical or the virtual robot, the participants were asked to complete a self-report questionnaire. As the virtual robot did not generate any noise, while the physical robot did, and because there was external background noise from the equipment and environment, we made the participants wear earplugs to neutralize most of the noise of the motors of the physical robot. Since auditory sensitivity was not the focus of our study, we excluded the auditory sensitivity from our evaluation. Before starting the experiment, we checked with each participant whether wearing the earplugs did not cancel out the vocal feedback of the robot (as described in Section 2). The selected movement (abduction of the left arm) is rather simple, but this movement is at present in use for arm rehabilitation, for example after stroke. Both physical and virtual robot did the movement at an average period of 5.6 s. The frequency was the same across all conditions. Trial repetition is fundamental for both the effectiveness of a rehabilitation therapy and the reliability of EEG measurements. Given the duration of the experiment (around 30 min for each participant), the simplicity of the movement further allowed us to keep the participants’ fatigue under control.
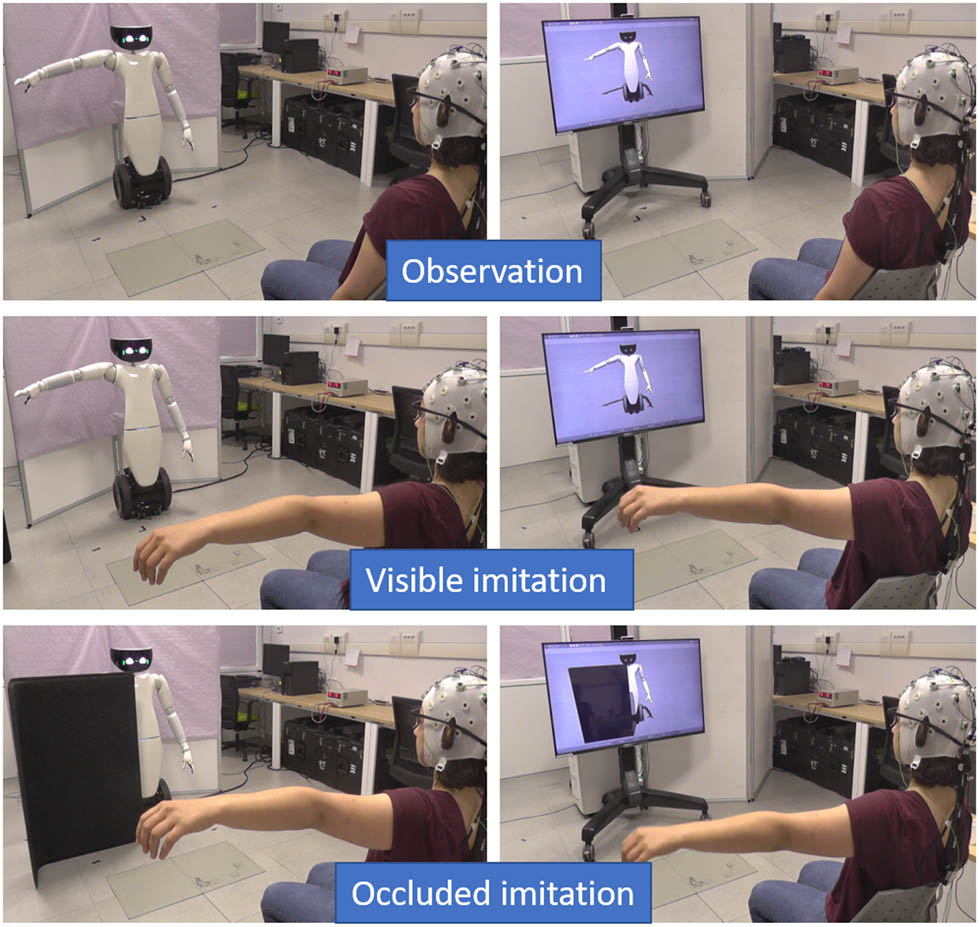
The experimental scenarios during observation, visible imitation, and occluded imitation (respectively, first, second, and third rows) for the physical robot and virtual robot (first and second columns, respectively).
2.2.4 Measurement
To evaluate the relationship between sensory sensitivity and the participants’ performance, we collected performance metrics and questionnaires described below.
2.2.4.1 Performance metrics
As described in Section 2, the framework recorded the participant skeleton and the “template” skeleton (see Figure 4). These recordings enabled comparing each participant’s performance in imitating the movement to the template during the visible imitation and occluded imitation conditions. We used the participant’s hand position as a predictor of good performance, as the hand’s movement is the widest compared to other key points involved in an abduction. As shown in Figure 4, the abduction movements (i.e., the arm is resting alongside the body and is then spread away from the midline of the body) occur mainly in the axes X and Y (perpendicular to the body and parallel to the body, respectively), and this is why we do not consider the Z axis in the measurements. As our framework does not enable us to guaranty the synchrony in the recording of the participants and the template, we corrected the lag between the signals. We computed the cross correlation between the participant and template’s hand position in the X and Y axes for each condition to determine the delay between two signals. We corrected the lag between two signals and computed their correlation coefficients, which indicates the “closeness” from one signal to another (see Figure 5). The correlation coefficient depends on the differences in the amplitude and the frequency of signals: a hand moving at a different velocity, frequency, or amplitude than the robot’s hand would produce a lower correlation coefficient.
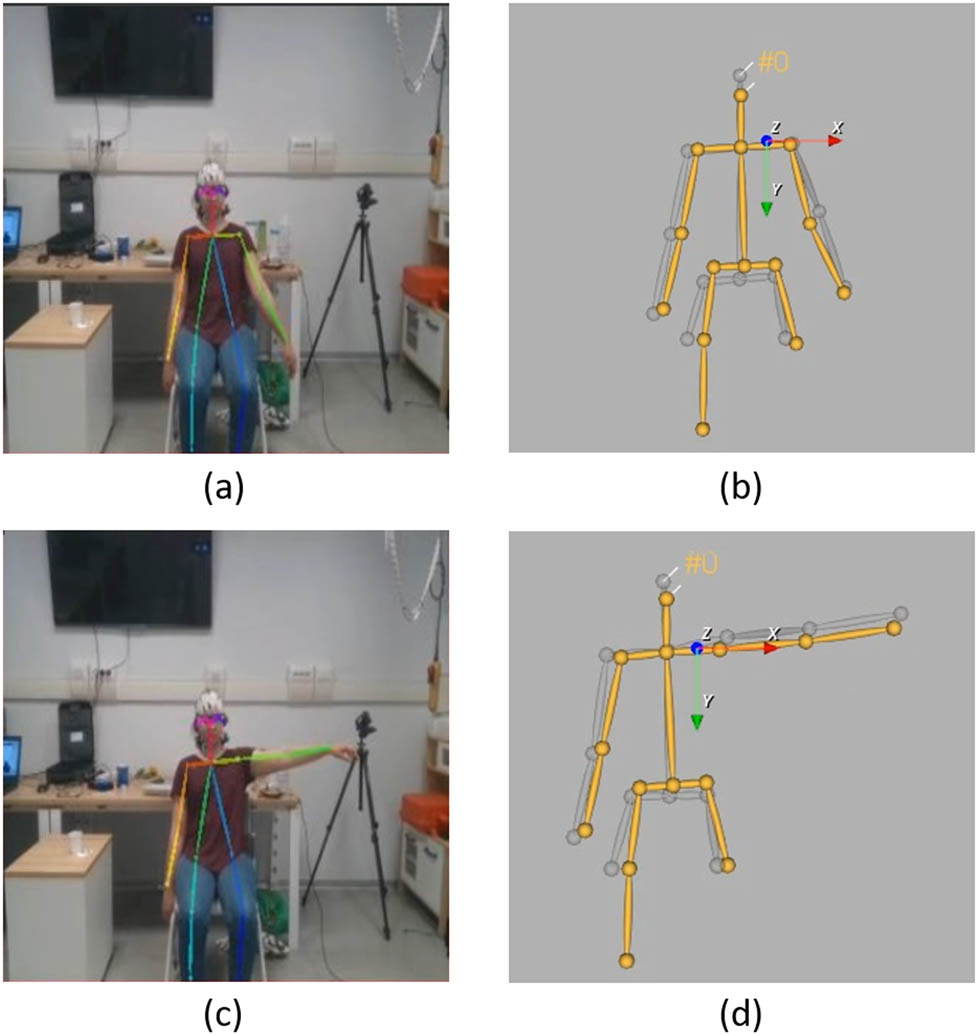
Captures of the participant’s and template’s skeletons during the abduction movement of the left arm. The movement occurs mainly in the X and Y axes (respectively, in red and green in (b) and (d)). The participant’s skeleton is tracked in the robot’s video feed as seen in (a) and (c). With the depth-sensor data and as explained in Section 2, the 3D skeleton of the participant, in yellow in (b) and (d), is extracted. The template’s skeleton is visible in gray in (b) and (c).
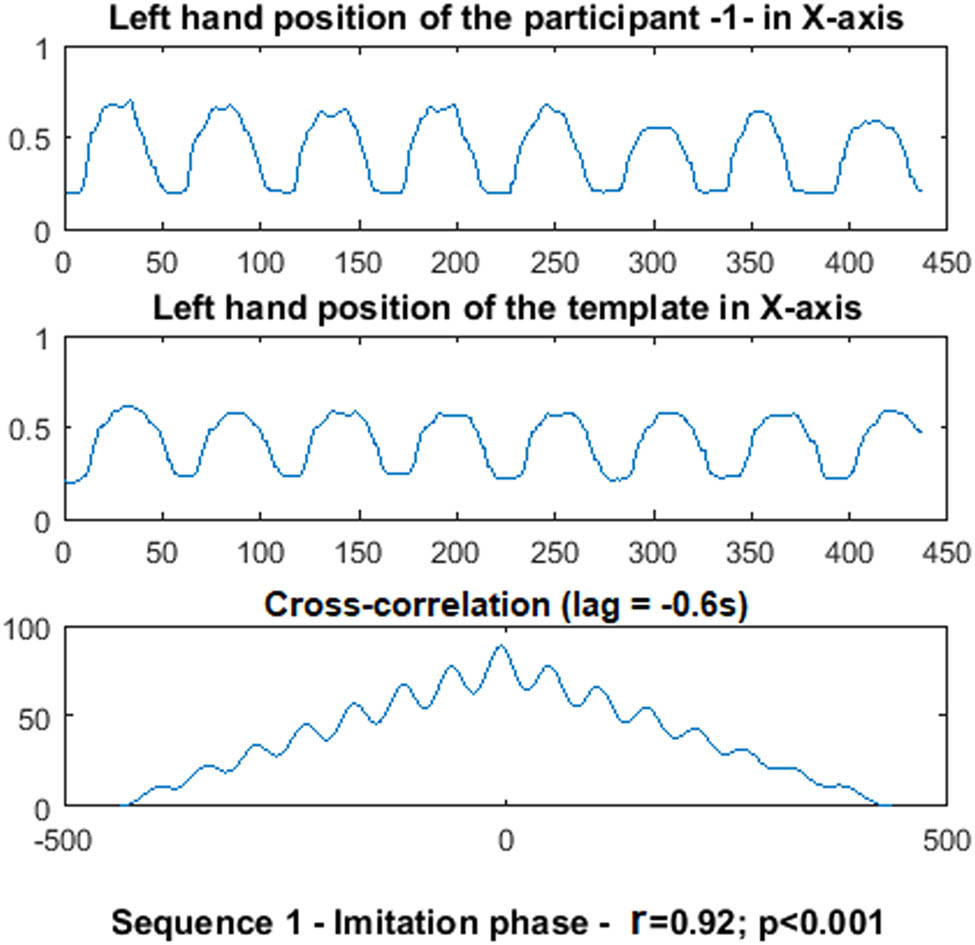
The template’s (top) and participant’s (middle) hand positions in the X axis during the imitation phase of the first sequence with the virtual robot. The cross correlation between the two signals is computed (bottom) and the lag between the two signals is corrected to compute the correlation coefficient (r) between the two signals. The correlation coefficient is taken as an indicator of good performance.
![Figure 6
Visualization of the interaction effect (robot embodiment × imitation condition) of the participants’ performance in the X axis with vision sensitivity. We used the median split to create a group of low-visual sensitivity (n = 6) and a group of high-visual sensitivity (n = 5; the remaining participants scored the median of 9, and therefore could not be grouped). Within-subject standard errors [31] were used for the error bars. This graph is purely for visualization purposes. Because of the small remaining group sizes, only the between-subject main effect of visual sensitivity group was significant, but none of the interactions anymore. We believe these graphs really show how the participants’ performance in X axis drops only if the virtual, but not if the physical, robot is occluded.](/https/www.degruyter.com/document/doi/10.1515/pjbr-2021-0014/asset/graphic/j_pjbr-2021-0014_fig_006.jpg)
Visualization of the interaction effect (robot embodiment × imitation condition) of the participants’ performance in the X axis with vision sensitivity. We used the median split to create a group of low-visual sensitivity (n = 6) and a group of high-visual sensitivity (n = 5; the remaining participants scored the median of 9, and therefore could not be grouped). Within-subject standard errors [31] were used for the error bars. This graph is purely for visualization purposes. Because of the small remaining group sizes, only the between-subject main effect of visual sensitivity group was significant, but none of the interactions anymore. We believe these graphs really show how the participants’ performance in X axis drops only if the virtual, but not if the physical, robot is occluded.
We computed the mean correlation score of each participant for the whole experiment per embodiment type (virtual robot; physical robot) and per condition (visible imitation; occluded imitation), resulting in each participant obtaining nine scores in both X and Y axes.
2.2.4.2 Sensory Perception Quotient (SPQ)
To screen the participants’ sensory sensitivity, we used the SPQ[2] [26]. This self-reported questionnaire enables determining one’s sensory sensitivity in five modalities: Hearing, Vision, Touch, Taste, and Smell. The questionnaire contains 92 items, and scoring them by subscales enables obtaining one’s sensitivity for each modality. The higher the score is, the more sensitive the person is. All participants filled out the questions only for the Vision, Hearing and Touch subscales of the SPQ (20 questions each). Each question can be answered by “strongly agree,” “agree,” “disagree,” and “strongly disagree.” The scoring key gives a table for each question corresponding to an answer, i.e., each question can score “0” or “1.” We compute for each subscale (Hearing, Visual, and Touch) the score corresponding to the sum of each question of a subscale. Therefore, each participant had a score between 0 and 20 for Visual, Hearing and Touch sensibility. The authors of the SPQ indicated that a higher score corresponds to a higher sensitivity, but they did not indicate ranges. The SPQ was originally developed for a population with ASD, to screen their sensory perception; however, the authors of the SPQ state that the questionnaire can be used for population with and without ASD [26]. We did not ask our participants to complete the Taste and Smell subscales, as these senses were not related to the experiment. However, as we used earplugs in our setup to cancel the high background and R1’s actuator noise, we did not use the Hearing subscale in our analysis as we are unsure on how it affected the participants’ hearing sensitivity.
2.2.4.3 Reported strategy questionnaire
After completing the six sequences of movements with the virtual and the physical robot, the participants were asked to describe what strategies they used to imitate the robot when the arm was occluded.
2.2.5 Data analysis
A 2 by 2 between-group analysis of covariance (ANCOVA) was conducted to assess the effect of the different robots on the participants’ performance in visible and occluded imitations. The independent variables were the type of robot (virtual, physical) and the imitation conditions (visible, occlusion). The dependent variable was the performance in the X and Y axes of the participants. The mean-centered visual sensitivity score from the SPQ was used as a covariate. We ran a similar ANCOVA but with mean-centered sensitivity to touch as a covariate instead of sensitivity to vision. In view of the results of the ANCOVA, we then computed bivariate (Pearson) correlations between the participants’ performance metrics in X and Y axes and their visual sensitivity score.
3 Results
3.1 Sensory sensitivity effects
On the X axis, there was a significant main effect of the robot’s embodiment (F(1, 14) = 7.81, p = 0.014, with a large effect size (partial η 2 = 0.358)) and of the imitation conditions (F(1, 14) = 8.50, p = 0.011, with a large effect size (partial η 2 = 0.378)). A significant interaction effect was also observed between the robot’s embodiment and the imitation conditions (F(1, 14) = 7.75, p = 0.015, with a large effect size (partial η 2 = 0.356)). These results suggest that both the robot’s embodiment and the imitation conditions impacted the participants’ performance on the X axis. See Figure 6 for a visual representation of the interaction effect with visual sensitivity. We followed up this analysis with correlations to observe the effect of the covariate (visual sensitivity score).
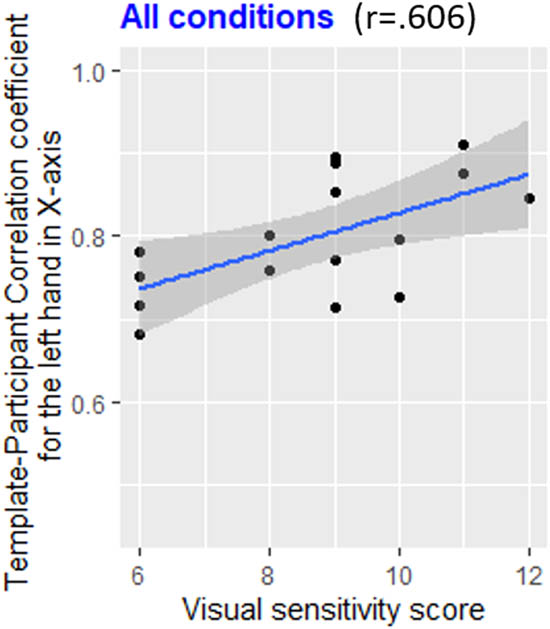
Scatterplot of the template–participant correlation coefficient for the left hand in the X axis in function of the participant’s visual sensitivity score for the whole experiment. The more a participant has a high-visual sensitivity score, the better the participant performed during the experiment.
We found significant relationships between the X axis of the participants’ performance and the visual sensitivity of the participants. First, we found that a higher visual sensitivity was positively correlated with a better performance of the participants over the whole experiment (r = 0.606, p = 0.0128, 95% CI [0.158, 0.847]; see Figure 7).

Scatterplot of the template–participant correlation coefficient for the left hand in the X axis in function of the participant’s visual sensitivity score for both embodiment types (physical – left and virtual – right). The more a participant has a high-visual sensitivity score, the better the participant performed when interacting with the virtual robot. This relationship was not found with the physical robot.
Regarding the embodiment types, we found that a higher visual sensitivity was positively correlated with a better performance of the participants when interacting with the virtual robot (r = 0.605, p = 0.0129, 95% CI [0.156, 0.847]), but we did not find any relationship in the physical robot condition (r = 0.188, p = ns, 95% CI [−0.339, 0.626]; see Figure 8).
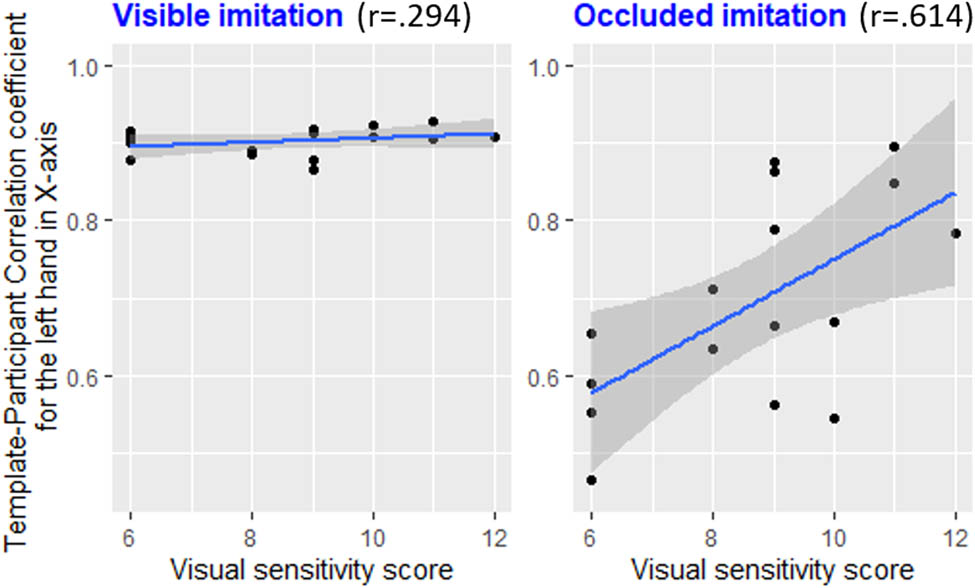
Scatterplot of the template–participant correlation coefficient for the left hand in the X axis in function of the participants visual sensitivity score, for both conditions (visible imitation – left and occluded imitation – right). The more a participant has a high-visual sensitivity score, the better the participant performed when performing the occluded imitation. This relationship was not found when the participant performed the visible imitation.
Regarding the condition of the task, we found that a higher visual sensitivity was positively correlated with a better performance of the participants in occluded imitation (r = 0.614, p = 0.0114, 95% CI [0.170, 0.851]), but we did not find any relationship for visible imitation (r = 0.294, p = ns, 95% CI [−0.236, 0.689]; see Figure 9).

Scatterplot of the template–participant correlation coefficient for the left hand in the X axis in function of the participants visual sensitivity score, for the interactions between the embodiment types (virtual and physical) and the conditions (visible imitation and occluded imitations). The more a participant has a high-visual sensitivity score, the better the participant performed when performing the occluded imitation with the virtual robot. The participants’ performance was not affected by their visual sensitivity in the other conditions.
Regarding the interactions between the embodiment types of the robot and the condition of the interaction, we only found that a higher visual sensitivity was positively correlated with better performance of the participants when interacting with the virtual robot when its arm was occluded (r = 0.607, p = 0.0126, 95% CI [0.160, 0.848]; see Figure 10).
We did not find any significant relationships between visual sensitivity and participants’ performance along the Y axis of the motion. The ANCOVA with sensitivity to touch yielded no significant main effect for sensitivity to touch in either X or Y axis. No significant interactions involving touch were observed. We did not find any significant relationships in the correlations between touch sensitivity and participants’ performance. The full results can be found in the Supplementary Materials.
3.2 Reported strategy questionnaire
Participants used different strategies to continue following the robot’s movement during the occluded imitation conditions. They reported a combination of strategies, involving the auditory and visual cues, delivered by the robot or internal processes such as counting or following their body rhythm (e.g., breathing). Table 2 provides some of the strategies reported by our participants. We categorized our participants’ strategies by classifying them as “auditory,” “visual,” or “internal” (see Table 3 for a contingency table of the participants’ strategies). When interacting with the physical robot, 5 of 16 participants reported to rely on visual cues, 10 on auditory cues, and 8 on internal processes. Ten of the participants used auditory cues even if they were wearing earplugs, as it did not neutralize all the sounds from the robot’s motors. During the interaction with the virtual robot, four participants reported having relied on visual cues, and all of them reported having relied on internal processes, such as counting or synchronizing with their breath to perform the task.
Examples of the participants’ strategies to continue following the robot’s movement during the occluded imitation conditions
Visual strategies | Physical robot |
“[I used the] reflection of the arm on its face”; “[I used] the vibrations of the right arm during the movement” | |
Virtual robot | |
“I used the shadow and the little movement of R1’s left arm”; “I used first the oscillation of [R1’s] body, that was lining towards the direction of the arm that was raising” | |
Auditory strategies | Physical robot |
“I was not seeing the robot’s arm but I was hearing its movement, so I followed its rhythm”; “I heard its mechanism” | |
Internal strategies | Physical robot |
“I counted the seconds when I was still able to see the movements, and I continued with the same rhythm”; “I was counting my breath and replicate the rhythm and number in the occluded part” | |
Virtual robot | |
“Creating a kind of metronome in my head for the movement and counting the repetitions”; “I remembered the rhythm between movements” |
Classification of the participants’ (N = 16) strategies as “auditory,” “visual,” or “internal” strategies when asked to imitate the physical and virtual robots during the occluded imitation condition. Participants may have used a combination of strategies to perform the task
Strategies | |||
---|---|---|---|
Agent | Auditory | Visual | Internal |
Physical robot | 10 | 5 | 8 |
Virtual robot | 0 | 4 | 16 |
4 Discussion
In this study, we evaluated the relationship between sensory sensitivity and the performance on an imitation task with a virtual and a physical robot. From the reported strategy questionnaire, we confirm that sensory strategies based on visual and hearing cues were used by the participants of our study alongside more internal strategies, like counting or synchronizing the movement with internal processes, like breathing. We observed that to perform the task with the virtual robot, the participants might have invested more cognitive effort as they all reported using counting or other internal processes to follow the movement, while only half of them used these strategies with the physical robot. Indeed, with the physical robot, participants reported to rely more on auditory and visual cues to perform the task, suggesting that the physical embodied robot provides indeed more physical presence than its virtual counterpart.
The participants’ performance in following the trajectory of the robot’s hand during the task showed a number of relationships with their visual sensitivity as scored with the SPQ questionnaire. Participants with a higher score in visual sensitivity performed the task in the X axis better across the entire experiment. However, this relationship between visual sensitivity score and performance was observed for interactions with the virtual robot, but not with the physical robot. We also found that participants with a higher sensitivity to vision performed better than those with lower sensitivity in the occluded imitation. Visual sensitivity did not play a role in the visible imitation condition. In sum, the visual sensitivity of participants was of benefit when performing occluded imitation with the virtual robot. Following on from these results, we propose that participants with a higher visual sensitivity might have been better able to detect subtle movements of the robot than participants with a lower sensitivity. For example, they could have been better able to detect the vibrations in the agent’s body, which were more subtle to detect on the virtual agent. These types of behaviors are indeed similar to the SPQ items that relate to visual screen sensitivity. This result is in line with the previous findings [3], where children diagnosed with ASD who rely more on visual cues were found to perform better in an imitation task.
Contrary to the X axis, we did not find similar results in the Y axis. Prior to the analysis of the data, we expected to find similar patterns in the X and Y axes, as the abduction movement of the arm is present and similar in both axes. We did not find an explanation to this difference in the participants’ performance in X and Y axes in the literature. However, this behavior can be explained by basic physics, as it is related to the forces and activation of the muscles applied to the arm during the abduction movement. Indeed, at the initial position (against the body) and the goal position (i.e., away from the body), the arm has a null velocity and therefore a null momentum (i.e., quantity of movement). At the goal position (i.e., away from the body), the arm is in extension and the muscles are activated. We hypothesize that the hand suffers less variation when the arm is stiffer, causing no difference in the variation in X and Y axes. Contrary, in the initial position, the arm is close to the resting position (i.e., the arm against the body). Thus, the participants had their relevant muscles passing from an active to a resting state in this position, inducing a wider variation in movement in the X axis compared to the Y axis. Furthermore, when the arm goes down, the Y component moves in favor of gravity, which might justify the lower variation too.
Finally, we did not find any significant relationship between the participants’ performance and touch sensitivity. The tangible nature of the robot’s embodiment did not appear to influence the performance of our participants depending on their touch sensitivity. However, touch was not involved in the task itself, so a lack of relationship with sensitivity to touch is not surprising.
Interestingly, visual information was discussed to be primordial in synchronization between two interaction partners. Indeed, during a repetitive task, two humans will synchronize [27,28]. As discussed in ref. [27], visual information of the movement enables partners to increase the synchrony. The authors asked participants to perform a puzzle while holding a pendulum and observed that when the visual stimuli was visible, the synchrony increased. Similarly, ref. [28] observed that people will synchronize with a stimulus displayed in the environment. However, in ref. [29], the authors discussed that in human–robot interaction, the synchronization does not emerge naturally. The robot needs to adapt to the human movements during the interaction, to retrieve this natural phenomenon. In our article, we did not record the difference in phase between the participants and the robots, but we nonetheless observed that visual cues (from the robot vibration, for example) could help in the imitation in frequency and amplitude in the occluded imitation phase, as there was no impact of occlusion on participants’ performance.
As participants’ performance in the physical robot condition was practically at ceiling, with no impact of occlusion, we suggest that a physical embodiment requires less cognitive strategies in continuing an imitation task under conditions with lack of sensory information, compared to its virtual counterpart. This is perhaps not surprising due to the larger amount of sensory cues that embodiment provides, even when the most relevant cue – the arm in our case – is unavailable. The interesting finding of our study is that people with higher visual sensitivity are able to keep a rhythm of imitation in the occluded condition (with virtual robot) better than those with lower visual sensitivity. This might be due to a better consolidation of sensorimotor representation of the task. Finally, it is also important to note that overall, the performance in the virtual occluded condition was substantially lower than the performance in the other conditions (physical robot and visible imitation, physical robot and occluded imitation, and virtual robot and visible imitation). This is due to a lack of additional sensory cues that the virtual agent’s embodiment provides, even in the absence of the most relevant sensory input. Hence, this speaks in favor of the idea that physical embodiment provides physical presence to a larger extent and allows for compensatory strategies, such as picking up additional cues and signals in the absence of the relevant channel.
Given the relationship we found, the absence of visual input might detrimentally affect a typical rehabilitation therapy. However, the quality of the therapy could benefit from the use of an embodied platform, specifically designed to enhance different sensory channels. Therefore, we believe that our findings are important when devising strategies for delivering rehabilitation. In the rehabilitation contexts, this should be taken into account when considering whether physical embodiment can be substituted only by virtual agents. For example, virtual agents are widely used to deliver rehabilitation therapies to elderly individuals; however, this population shows a degradation in their visual functions [30]. The use of physical robot may be beneficial for them in view of our results.
A limitation of our work is the difference in size of both robots. The virtual robot was indeed smaller than the real robot, as it is shown in Figure 3. It was already discussed that the size of virtual agent against real artificial agent could play a role in the perception and interaction with the agent (see ref. [19]). Indeed, this review suggests that people respond more favorably to real agent than virtual agents. We also obtained these results in our previous paper [17]. We cannot exclude this effect to happen in our experiment. However, we made sure that during the task, the movement of the robot was clearly visible in both condition. Another limitation of our work is the subject pool number, as we recruited only 16 participants. This was due to the limited time of the availability of the robot and the long duration the experiment and installation of the participants.
To our knowledge, this is the first study investigating the relations between sensory sensitivity and the performance in an imitation task with a real or a virtual robot. Taken together, we show that visual sensitivity predicts performance in an imitation task, especially in the absence of complete sensory input (as was the case for our virtual robot condition, with occlusion). Even though these results are only preliminary and need to be replicated with larger samples, including patients, they can be informative with respect to designing human–robot interaction for rehabilitation. These results should be followed up with studies with clinical populations, such as children diagnosed with ASD who show wider and more pronounced interindividual variability in sensory sensitivity [6] and elderly people suffering from sensitivity impairments (e.g., hearing or vision loss) due to aging conditions.
Acknowledgments
The project leading to these results has received funding from the European Union’s Horizon 2020 research and innovation program under the Marie Skłodowska-Curie grant agreement no. 754490 – MINDED project and by the Joint Lab between IIT and Fondazione Don Carlo Gnocchi Onlus (Valerio Gower and Furio Gramatica). The authors thank Nina Hinz and Serena Marchesi for their assistance with data collection.
The content of this work reflects the author’s view only and the EU Agency is not responsible for any use that may be made of the information it contains.
-
Data availability statement: The datasets generated during and/or analysed during the current study are available from the corresponding author on reasonable request.
Appendix
Instruction to the participants
The following instructions were provided by the experimenters to the participants:
The robot will demonstrate a simple exercise. Please just observe and try to sit still. When the robot says “Now do the same with me,” try to copy the same motion together with the robot, using your left arm. Then we will put a panel between you and the robot’s arm, so you can’t see it anymore. It is very important that you keep looking at the robot: try not to be distracted by the placement of the panel. Even when you can’t see the robot’s arm anymore, try to keep copying the same motion.
After this exercise, please take a break for as long as you need. When you indicate that you’re ready, we will repeat the exercise, followed by another break until we have completed the exercise six times. During each break, you can take as long as you need. If you feel any discomfort, please stop immediately. This has no consequences for your participation or your payment.
After these exercises, you can have a rest while we change the setup. When you’re ready, we will do the same but this time via the screen/physical robot
To reiterate:
Try to remain still when you’re just observing the robot. When you’re copying the robot, try to only move your left/right arm.
You decide how long your breaks are.
You can stop at any time.
Additional results
Plots of the participants’ performance (i.e., the cross correlation between the participant and template’s hand position in X axes, R) depending on their first interaction partner (physical or virtual robot).
Sensory sensitivity effects on the cross correlation between the participant and template’s hand position in the observed axes.
(a) ANCOVA with sensitivity to vision as covariate in X axis
Condition F(1,14) p Partial η 2 Robot 7.81 0.014* 0.358 Imitation 8.50 0.011* 0.378 Robot × imitation 7.75 0.015* 0.356 (b) Correlations between the X axis of the participants’ performance and the visual sensitivity of the participants.
Condition R p 95%CI All conditions 0.606 0.013* [0.158, 0.847] Physical robot 0.189 0.484 [−0.339, 0.626] Virtual robot 0.605 0.013* [0.156, 0.847] Visible imitation 0.294 0.268 [−0.236, 0.689] Occluded imitation 0.614 0.011* [0.170, 0.851] Physical robot × visible imitation 0.126 0.641 [−0.394, 0.585] Physical robot × occluded imitation 0.187 0.488 [−0.340, 0.625] Virtual robot × visible imitation 0.373 0.154 [−0.150, 0.733] Virtual robot × occluded imitation 0.607 0.013* [0.150, 0.848] (c) ANCOVA with sensitivity to vision as covariate in Y axis
Condition F(1,14) p Partial η 2 Robot 2.59 0.130 0.156 Imitation 3.52 0.082 0.201 Robot × imitation 3.71 0.750 0.209 (d) Correlations between the Y axis of the participants’ performance and the visual sensitivity of the participants.
Condition R p 95%CI All conditions 0.310 0.243 [−0.219, 0.698] Physical robot −0.392 0.133 [−0.743, 0.129] Virtual robot 0.353 0.180 [−0.173, 0.722] Visible imitation −0.141 0.602 [−0.595, 0.381] Occluded imitation 0.375 0.152 [−0.148, 0.734] Physical robot × visible imitation −0.288 0.280 [−0.686, 0.243] Physical robot × occluded imitation −0.473 0.064 [−0.785, 0.030] Virtual robot × visible imitation −0.073 0.788 [−0.549, 0.439] Virtual robot × occluded imitation 0.401 0.124 [−0.118, 0.748] (e) ANCOVA with sensitivity to touch as covariate in X axis
Condition F(1,14) p Partial η 2 Robot 0.032 0.862 0.020 Imitation 0.000 0.990 0.000 Robot × imitation 0.013 0.912 0.001 (f) Correlations between the X axis of the participants’ performance and the touch sensitivity of the participants.
Condition R p 95%CI All conditions −0.086 0.751 [−0.558, 0.428] Physical robot −0.214 0.426 [−0.642, 0.315] Virtual robot −0.068 0.803 [−0.545, 0.443] Visible imitation −0.363 0.167 [−0.728, 0.162] Occluded imitation −0.045 0.869 [−0.529, 0.461] Physical robot × visible imitation −0.330 0.211 [−0.710, 0.198] Physical robot × occluded imitation −0.048 0.860 [−0.531, 0.459] Virtual robot × visible imitation −0.342 0.194 [−0.716, 0.185] Virtual robot × occluded imitation −0.042 0.877 [−0.527, 0.463] (g) ANCOVA with sensitivity to touch as covariate in Y axis
Condition F(1,14) p Partial η 2 Robot 0.070 0.795 0.005 Imitation 0.047 0.832 0.003 Robot × imitation 0.031 0.863 0.002 (h) Correlations between the Y axis of the participants’ performance and the Touch sensitivity of the participants.
Condition | R | p | 95%CI |
---|---|---|---|
All conditions | −0.008 | 0.978 | [−0.501, 0.490] |
Physical robot | −0.436 | 0.092 | [−0.766, 0.077] |
Virtual robot | 0.031 | 0.910 | [−0.472, 0.519] |
Visible imitation | −0.160 | 0.554 | [−0.607, 0.365] |
Occluded imitation | 0.022 | 0.937 | [−0.479, 0.512] |
Physical robot × visible imitation | −0.436 | 0.091 | [−0.766, 0.076] |
Physical robot × occluded imitation | −0.394 | 0.131 | [−0.745, 0.126] |
Virtual robot × visible imitation | −0.044 | 0.872 | [−0.528, 0.462] |
Virtual robot × occluded imitation | 0.041 | 0.881 | [−0.464, 0.526] |
References
[1] S. Andrist , B. Mutlu , and A. Tapus , “Look like me: Matching robot personality via gaze to increase motivation,” in Proceedings of the 33rd Annual ACM Conference on Human Factors in Computing Systems, New York, NY, USA, 2015, pp. 3603–3612, https://doi.org/10.1145/2702123.2702592 .10.1145/2702123.2702592Search in Google Scholar
[2] L. Robert , “Personality in the human robot interaction literature: A review and brief critique,” in Proceedings of the 24th Americas Conference on Information Systems, Aug 16–18, New Orleans, LA. Available at SSRN: https://ssrn.com/abstract=3308191. [Accessed: Sep. 18, 2019].Search in Google Scholar
[3] P. Chevalier , G. Raiola , J. C. Martin , B. Isableu , C. Bazile , and A. Tapus , “Do sensory preferences of children with autism impact an imitation task with a robot?,” in Proceedings of the 2017 ACM/IEEE International Conference on Human-Robot Interaction, New York, NY, USA, 2017, pp. 177–186, https://doi.org/10.1145/2909824.3020234 .10.1145/2909824.3020234Search in Google Scholar
[4] R. Agrigoroaie and A. Tapus , “Influence of robot’s interaction style on performance in a Stroop task,” in ICSR 2017: Social Robotics, Lecture Notes in Computer Science, vol. 10652, A. Kheddar et al. (Eds.), Cham: Springer, 2017, pp. 95–104, https://doi.org/10.1007/978-3-319-70022-9_10 10.1007/978-3-319-70022-9_10Search in Google Scholar
[5] D. Shepherd , M. Heinonen-Guzejev , M. J. Hautus , and K. Heikkilä , “Elucidating the relationship between noise sensitivity and personality,” Noise Health, vol. 17, no. 76, pp. 165–171, 2015, https://doi.org/10.4103/1463-1741.155850 .10.4103/1463-1741.155850Search in Google Scholar PubMed PubMed Central
[6] APA , Diagnostic and Statistical Manual of Mental Disorders (DSM-5®), American Psychiatric Pub, 2013.Search in Google Scholar
[7] J. Ward , “Individual differences in sensory sensitivity: a synthesizing framework and evidence from normal variation and developmental conditions,” Cogn. Neurosci., vol. 10, no. 3, pp. 139–157, 2019, https://doi.org/10.1080/17588928.2018.1557131 .10.1080/17588928.2018.1557131Search in Google Scholar PubMed
[8] A. E. Robertson and D. R. Simmons , “The relationship between sensory sensitivity and autistic traits in the general population,” J. Autism Dev. Disord., vol. 43, pp. 775–784, 2013, https://doi.org/10.1007/s10803-012-1608-7 .10.1007/s10803-012-1608-7Search in Google Scholar PubMed
[9] E. N. Aron and A. Aron , “Sensory-processing sensitivity and its relation to introversion and emotionality,” J. Pers. Soc. Psychol., vol. 73, no. 2, pp. 345–368, 1997, https://doi.org/10.1037/0022-3514.73.2.345 .10.1037/0022-3514.73.2.345Search in Google Scholar
[10] P. Chevalier , J.-C. Martin , B. Isableu , C. Bazile , and A. Tapus , “Impact of sensory preferences of individuals with autism on the recognition of emotions expressed by two robots, an avatar, and a human,” Auton. Robot., vol. 41, pp. 613–635, 2017, https://doi.org/10.1007/s10514-016-9575-z .10.1007/s10514-016-9575-zSearch in Google Scholar
[11] P. Chevalier , J.-C. Martin , B. Isableu , C. Bazile , D.-O. Iacob , and A. Tapus , “Joint attention using human-robot interaction: Impact of sensory preferences of children with autism,” in 2016 25th IEEE International Symposium on Robot and Human Interactive Communication (RO-MAN), New York, NY, 2016, pp. 849–854, https://doi.org/10.1109/ROMAN.2016.7745218 .10.1109/ROMAN.2016.7745218Search in Google Scholar
[12] R. Wilk and M. J. Johnson , “Usability feedback of patients and therapists on a conceptual mobile service robot for inpatient and home-based stroke rehabilitation,” in 5th IEEE RAS/EMBS International Conference on Biomedical Robotics and Biomechatronics, Sao Paulo, 2014, pp. 438–443, https://doi.org/10.1109/BIOROB.2014.6913816 .10.1109/BIOROB.2014.6913816Search in Google Scholar
[13] K. Winkle , P. Caleb-Solly , A. Turton , and P. Bremner , “Social robots for engagement in rehabilitative therapies: Design implications from a study with therapists,” in Proceedings of the 2018 ACM/IEEE International Conference on Human-Robot Interaction, Chicago, IL, USA, 2018, pp. 289–297, https://doi.org/10.1145/3171221.3171273 .10.1145/3171221.3171273Search in Google Scholar
[14] A. Tapus , C. Ţăpuş , and M. J. Matarić , “User–robot personality matching and assistive robot behavior adaptation for post-stroke rehabilitation therapy,” Intell. Serv. Robot., vol. 1, art. 169, 2008, https://doi.org/10.1007/s11370-008-0017-4 .10.1007/s11370-008-0017-4Search in Google Scholar
[15] B. Scassellati , H. Admoni , and M. Matarić , “Robots for use in autism research,” Ann. Rev. Biomed. Eng., vol. 14, pp. 275–294, 2012, https://doi.org/10.1146/annurev-bioeng-071811-150036 .10.1146/annurev-bioeng-071811-150036Search in Google Scholar PubMed
[16] A. Parmiggiani , L. Fioro , A. Scalzo , A. V. Sureshbabu , M. Randazzo , M. Maggiali, et al., “The design and validation of the R1 personal humanoid,” in 2017 IEEE/RSJ International Conference on Intelligent Robots and Systems (IROS), Vancouver, BC, 2017, pp. 674–680, https://doi.org/10.1109/IROS.2017.8202224 .10.1109/IROS.2017.8202224Search in Google Scholar
[17] V. Vasco , C. Willemse , P. Chevalier , D. De Tommaso , V. Gower , F. Gramatica , et al., “Train with me: A study comparing a socially assistive robot and a virtual agent for a rehabilitation task,” in ICSR 2019: Social Robotics, Lecture Notes in Computer Science, vol. 11876, M. Salichs et al. (Eds.). Cham: Springer, 2019, pp. 453–463, https://doi.org/10.1007/978-3-030-35888-4_42 .10.1007/978-3-030-35888-4_42Search in Google Scholar
[18] E. Deng , B. Mutlu , and M. J. Mataric , Embodiment in Socially Interactive Robots, Now Foundations and Trends, 2019, https://doi.org/10.1561/2300000056 .10.1561/9781680835472Search in Google Scholar
[19] J. Li , “The benefit of being physically present: A survey of experimental works comparing copresent robots, telepresent robots and virtual agents,” Int. J. Hum. Comput. Stud., vol. 77, pp. 23–37, 2015, https://doi.org/10.1016/j.ijhcs.2015.01.001 .10.1016/j.ijhcs.2015.01.001Search in Google Scholar
[20] G. Metta , P. Fitzpatrick , and L. Natale , “YARP: Yet another robot platform,” Int. J. Adv. Robot. Syst., vol. 3, no. 1, 2006, https://doi.org/10.5772/5761 .10.5772/5761Search in Google Scholar
[21] Z. Cao , G. Hidalgo , T. Simon , S.-E. Wei , and Y. Sheikh , “OpenPose: Realtime multi-person 2D pose estimation using part affinity fields,” arXiv:1812.08008, 2018. [Accessed: Sep. 18, 2019].10.1109/TPAMI.2019.2929257Search in Google Scholar PubMed
[22] R. Bertani , C. Melegari , M. C. De Cola , A. Bramanti , P. Bramanti , and R. S. Calabrò , “Effects of robot-assisted upper limb rehabilitation in stroke patients: A systematic review with meta-analysis,” Neurol. Sci., vol. 38, pp. 1561–1569, 2017, https://doi.org/10.1007/s10072-017-2995-5 .10.1007/s10072-017-2995-5Search in Google Scholar PubMed
[23] J. C. Pulido , J. C. González , C. Suárez-Mejías , A. Bandera , P. Bustos , and F. Fernández , “Evaluating the child–robot interaction of the NAO therapist platform in pediatric rehabilitation,” Int. J. Soc. Robot., vol. 9, pp. 343–358, 2017, https://doi.org/10.1007/s12369-017-0402-2 .10.1007/s12369-017-0402-2Search in Google Scholar
[24] B. Robins , P. Dickerson , P. Stribling , and K. Dautenhahn , “Robot-mediated joint attention in children with autism: A case study in robot-human interaction,” Interact. Stud., vol. 5, no. 2, pp. 161–198, 2004.10.1075/is.5.2.02robSearch in Google Scholar
[25] S. Schneider , B. Wrede , C. Cifuentes , S. S. Griffiths , and S. Wermter , “PREC 2018: Personal robots for exercising and coaching,” in Companion of the 2018 ACM/IEEE International Conference on Human-Robot Interaction, New York, NY, USA, 2018, pp. 401–402, https://doi.org/10.1145/3173386.3173566 .10.1145/3173386.3173566Search in Google Scholar
[26] T. Tavassoli , R. A. Hoekstra , and S. Baron-Cohen , “The sensory perception quotient (SPQ): Development and validation of a new sensory questionnaire for adults with and without autism,” Mol. Autism, vol. 5, art. 29, 2014, https://doi.org/10.1186/2040-2392-5-29 .10.1186/2040-2392-5-29Search in Google Scholar PubMed PubMed Central
[27] M. J. Richardson , K. L. Marsh , and R. C. Schmidt , “Effects of visual and verbal interaction on unintentional interpersonal coordination,” J. Exp. Psychol. Hum. Percept. Perform., vol. 31, no. 1, pp. 62–79, 2005, https://doi.org/10.1037/0096-1523.31.1.62 .10.1037/0096-1523.31.1.62Search in Google Scholar PubMed
[28] R. C. Schmidt , M. J. Richardson , C. Arsenault , and B. Galantucci , “Visual tracking and entrainment to an environmental rhythm,” J. Exp. Psychol. Hum. Percept. Perform., vol. 33, no. 4, pp. 860–870, 2007, https://doi.org/10.1037/0096-1523.33.4.860 .10.1037/0096-1523.33.4.860Search in Google Scholar PubMed
[29] T. Lorenz , A. Weiss , and S. Hirche , “Synchrony and reciprocity: Key mechanisms for social companion robots in therapy and care,” Int. J. Soc. Robot., vol. 8, pp. 125–143, 2016, https://doi.org/10.1007/s12369-015-0325-8 .10.1007/s12369-015-0325-8Search in Google Scholar
[30] N. S. Majid , N. E. Badarudin , and N. A. Yahaya , “The awareness of visual function deterioration in the elderly: A review,” J. Optom. Eye Health Res., vol. 1, no. 1, pp. 18–33, 2019. Available: http://mymedr.afpm.org.my/publications/79842. [Accessed: Aug. 25, 2020].Search in Google Scholar
[31] G. R. Loftus and M. E. J. Masson , “Using confidence intervals in within-subject designs,” Psychon. Bull. Rev., vol. 1, pp. 476–490, 1994, https://doi.org/10.3758/BF03210951 .10.3758/BF03210951Search in Google Scholar PubMed
© 2021 Pauline Chevalier et al., published by De Gruyter
This work is licensed under the Creative Commons Attribution 4.0 International License.